Computer Simulations Modeling the Future Unveiling Possibilities
Computer simulations modeling the future offer a fascinating glimpse into potential scenarios. From predicting market fluctuations to understanding climate change impacts, these powerful tools are transforming how we approach complex challenges. This exploration delves into the various types of simulations, their applications across diverse fields, the importance of data, and the ethical considerations surrounding their use. We’ll examine agent-based modeling, system dynamics, and Monte Carlo simulations, seeing how they’re used in economics, climate science, and healthcare.
Building accurate models requires meticulous attention to data quality and validation. The insights gleaned from these simulations can inform crucial decisions, shaping policies and strategies in a rapidly changing world. Understanding the strengths and weaknesses of different simulation methods is vital for effective application and accurate interpretation of results.
Introduction to Future Modeling with Simulations
Computer simulations are powerful tools for exploring and understanding complex systems, especially when predicting future scenarios. They allow us to test hypotheses, model various factors, and visualize potential outcomes without the constraints of real-world experimentation. This approach is invaluable for understanding long-term trends and making informed decisions in areas like climate change, resource management, and economic forecasting. The power of simulation lies in its ability to capture intricate interactions between variables and create dynamic models of how these interactions might play out over time.Future modeling simulations often use a combination of quantitative data and qualitative insights.
The simulations themselves don’t “predict” the future in a deterministic way; rather, they offer a range of plausible outcomes based on different scenarios and inputs. This approach is crucial because the future is inherently uncertain, and models can help us understand the possible consequences of different actions and choices.
Different Types of Computer Simulations for Future Predictions
Computer simulations for future predictions employ various techniques. Agent-based modeling focuses on the interactions between individual agents, each with their own set of rules and behaviors. This approach is useful for modeling complex social systems, such as the spread of diseases or the evolution of markets. System dynamics models represent the interactions between different parts of a system, enabling analysis of feedback loops and long-term trends.
Monte Carlo simulations use random sampling to model uncertainty and variability in inputs, generating a range of possible outcomes. These methods are often combined to provide a more comprehensive understanding of the future.
Steps Involved in Building a Computer Simulation Model
Building a simulation model involves several key steps. First, a clear understanding of the system being modeled is essential. This involves identifying the key variables, their relationships, and the constraints they face. Second, a suitable simulation methodology must be chosen, considering the complexity of the system and the desired level of detail. Third, data collection and input parameters are crucial, as the accuracy of the model hinges on these inputs.
Next, the model is developed and tested. Finally, the model is validated and refined to ensure accuracy and reliability.
Computer simulations are crucial for predicting future scenarios, but what happens when the underlying hardware limitations of traditional silicon-based chips start to constrain their power? This directly impacts our ability to model complex systems accurately. The future of powerful simulations depends on breakthroughs in areas like life after Moore’s Law beyond silicon , which will drive innovation in materials science and computing architecture.
Ultimately, these advancements will be essential for pushing the boundaries of what computer simulations can achieve.
Limitations and Potential Biases in Future Modeling Simulations
Simulation models, while powerful, are not without limitations. One significant limitation is the inherent uncertainty of future events. The accuracy of simulations depends on the accuracy of the data used to create the model, the validity of the assumptions made, and the complexity of the system being modeled. Another important concern is the potential for biases in the data or assumptions.
These biases can lead to inaccurate or misleading predictions. Finally, the complexity of many real-world systems often makes it difficult to fully capture all relevant factors and interactions in a simulation model.
Comparison of Simulation Methods
Method | Strengths | Weaknesses | Assumptions |
---|---|---|---|
Agent-Based Modeling | Captures individual interactions, complex social dynamics, and emergent behavior. Useful for simulating systems with many interacting components. | Can be computationally intensive, requires detailed knowledge of individual agent behavior, and may be difficult to validate. | Individual agent behavior, rules of interaction, initial conditions. |
System Dynamics | Effective at representing feedback loops and long-term trends in complex systems, allowing for exploration of system dynamics and identifying tipping points. | May struggle with non-linear or chaotic systems, may not capture the variability of individual agents. | Relationships between variables, feedback loops, system parameters. |
Monte Carlo Simulation | Excellent for modeling uncertainty and variability in inputs, generating a range of possible outcomes and providing probability distributions. | Can be computationally expensive for large numbers of variables, and may not fully capture complex dependencies between variables. | Probability distributions for inputs, independence of random variables. |
Applications in Various Fields
Computer simulations are proving increasingly valuable tools for understanding and predicting future trends across diverse disciplines. From forecasting economic fluctuations to modeling the impacts of climate change, these digital experiments offer a powerful means to explore complex systems and potentially mitigate risks. By creating virtual representations of real-world phenomena, researchers can test hypotheses, analyze scenarios, and ultimately inform policy decisions.The power of simulation lies in its ability to isolate variables, manipulate parameters, and observe the cascading effects of change.
This iterative process of testing and refinement allows for a deeper understanding of cause-and-effect relationships, which is crucial for anticipating future challenges and opportunities in various fields.
Economic Applications
Economic models often rely on intricate interactions between individuals and market forces. Agent-based simulations provide a framework for understanding these interactions. These models track the decisions and behaviors of numerous “agents,” which can represent consumers, businesses, or even entire industries. By simulating various scenarios, researchers can analyze the impact of factors like policy changes, technological advancements, or shifts in consumer preferences on market fluctuations.
For instance, agent-based models have been employed to simulate the effects of different tax policies on consumer spending or the spread of innovation within a market.
Climate Science Applications
Climate science faces the daunting task of predicting the complex and multifaceted effects of human activities on the planet. System dynamics models, which consider the interconnectedness of various components of the climate system, offer valuable insights. These models simulate the interactions between factors like greenhouse gas emissions, ocean temperatures, and ice sheet melt, enabling researchers to project potential future climate scenarios.
A notable example involves modeling the impact of rising CO2 levels on global temperatures and sea levels, enabling researchers to assess the potential consequences of inaction on climate change mitigation.
Healthcare Applications
Healthcare simulations often employ Monte Carlo methods to analyze probabilistic outcomes. These models account for various uncertainties and random events, such as patient variability or the unpredictable spread of infectious diseases. Monte Carlo simulations, with their probabilistic nature, can help estimate the likelihood of different outcomes related to disease spread, treatment effectiveness, or resource allocation. For example, these simulations have been used to model the potential spread of pandemics, allowing researchers to evaluate the effectiveness of various intervention strategies, such as social distancing measures or vaccination campaigns.
Table of Simulation Applications
Field | Simulation Type | Application | Example |
---|---|---|---|
Economics | Agent-based | Market fluctuations, policy analysis | Simulating the impact of a carbon tax on energy markets |
Climate Science | System dynamics | Global warming effects, climate change mitigation | Projecting future sea-level rise due to glacial melt |
Healthcare | Monte Carlo | Disease spread, treatment efficacy | Estimating the effectiveness of different antiviral treatments in containing a pandemic |
Data Sources and Model Inputs
Building accurate future models relies heavily on the quality and comprehensiveness of the data used as input. The choice of data sources, their validation, and the subsequent impact on model accuracy are critical considerations in the development of any reliable simulation. Without robust data, even the most sophisticated algorithms can produce unreliable and misleading projections.The foundation of any future model rests on its input data.
The accuracy and reliability of the simulation’s output directly correlate with the quality of the input data. Carefully selected and meticulously validated data sources form the bedrock upon which credible predictions are built. This section delves into the crucial role of data sources, emphasizing their importance in shaping the accuracy and reliability of future simulations.
Importance of Data in Simulations
Accurate computer simulations require comprehensive and reliable data. The quality of the input data directly affects the accuracy of the output, influencing the reliability of the predictions. Data must be relevant to the specific questions the simulation seeks to answer. Data validation and cleaning procedures are essential to minimize errors and ensure the model’s integrity.
Different Data Sources in Future Modeling
Various data sources contribute to future modeling simulations. These sources encompass a wide spectrum of information, each contributing unique insights. The choice of data sources depends on the specific application and the nature of the questions being addressed.
- Historical Data: Past trends and patterns are crucial for understanding historical context. Historical data provides a baseline against which future projections can be evaluated. Examples include economic indicators, population statistics, climate records, and technological advancements over time. The use of historical data allows for the identification of potential future trends and patterns. Analyzing historical data enables the identification of correlations and causal relationships that can be incorporated into the model.
- Real-time Data: Current conditions and events significantly impact future projections. Data from sensors, social media, and financial markets provide up-to-the-minute insights into the present state of affairs. This real-time data stream allows the simulation to adapt to current conditions and adjust projections based on emerging trends. For instance, stock market data can be used to assess economic conditions and adjust predictions accordingly.
- Expert Opinions and Forecasts: Expert opinions and forecasts provide valuable contextual information. Experts in specific fields offer valuable insights based on their knowledge and experience. This can be particularly useful in areas where historical data is limited or where complex interactions are difficult to quantify. Examples include climate scientists, economists, and sociologists. Their opinions help shape the model’s assumptions and guide its development.
Computer simulations are fascinating tools for predicting future scenarios, but the real world can sometimes surprise us. Consider the recent legislation, like the new bill making file swapping a felony , which highlights the unpredictable ways laws can impact technology and society. These unexpected twists don’t necessarily invalidate the value of computer simulations, but they do remind us that future predictions are always a blend of calculated possibilities and unforeseen variables.
- Scenario Planning Data: Hypothetical situations and alternative futures are vital for understanding the potential impact of various factors. Scenario planning data explores various possibilities and their potential outcomes, offering insights into the range of possible futures. This includes projections under different economic scenarios, environmental conditions, and technological advancements.
Data Validation and Cleaning
Validating and cleaning data is essential for the accuracy and reliability of computer simulations. Inaccurate or incomplete data can lead to flawed results, affecting the overall validity of the simulation. Data cleaning techniques, such as identifying and correcting errors, inconsistencies, and missing values, ensure the integrity of the data used in the model.
- Data Cleaning Techniques: Data cleaning techniques aim to improve data quality by addressing inconsistencies, errors, and missing values. Common techniques include outlier detection, imputation of missing data, and data transformation. For instance, inconsistent data formats can be standardized, and outliers identified and removed.
- Validation Methods: Data validation methods confirm the accuracy and reliability of the data. These methods include comparing data to known values, checking for logical inconsistencies, and assessing the internal consistency of the data. This process ensures the reliability of the data. For example, cross-checking historical population data against census records can validate the accuracy of the data.
Evaluating Data Quality
Assessing the quality of data is crucial for ensuring the reliability of simulations. Different metrics can be used to evaluate the quality of data. These metrics include data accuracy, completeness, consistency, and timeliness.
- Accuracy and Completeness: The accuracy and completeness of data are fundamental aspects of data quality. Accurate data reflects reality, while complete data covers all relevant aspects. Accuracy and completeness directly affect the model’s ability to capture real-world phenomena and project future trends.
- Consistency and Timeliness: Consistency and timeliness are equally important. Consistent data ensures that the data is uniform across different sources and periods. Timely data allows the model to incorporate recent developments and changes. Inconsistencies and delays can undermine the model’s effectiveness.
Impact of Data Types on Simulation Accuracy
Different data types have varying impacts on the accuracy of simulations. Quantitative data, such as economic indicators or population figures, allows for precise calculations and estimations. Qualitative data, such as expert opinions or subjective assessments, can provide contextual information and nuance. The interplay of these data types shapes the model’s understanding of the future. A balanced approach incorporating both types of data leads to more robust and comprehensive simulations.
Output Interpretation and Visualization: Computer Simulations Modeling The Future
Turning raw simulation data into actionable insights is crucial for future modeling. Visualizing results in a clear and intuitive manner allows us to identify patterns, trends, and potential risks or opportunities. Effective visualization empowers stakeholders to understand complex simulations and make informed decisions based on the predicted future scenarios. This process, however, requires careful consideration of uncertainty and sensitivity, ensuring the presentation of results is transparent and trustworthy.
Different Visualization Techniques
Various techniques can effectively represent simulation outputs. Choosing the right approach depends on the nature of the data and the insights we want to extract. Different visualization methods highlight different aspects of the simulation results.
- Charts: Charts are versatile for displaying relationships between variables, such as trends over time or comparisons across categories. Line charts are excellent for showing trends in data over time, while bar charts can be used to compare data points across different categories. For example, a line chart showing the projected growth of a city’s population over the next 50 years can easily reveal potential challenges in infrastructure planning.
- Graphs: Graphs provide a visual representation of complex relationships between variables. Scatter plots are particularly useful for identifying correlations between two or more variables. For instance, a scatter plot illustrating the relationship between GDP growth and carbon emissions can reveal potential trade-offs or dependencies.
- Maps: Maps are invaluable for geographically distributed data. They can visualize the spatial distribution of factors, such as population density or resource availability. A map showing the predicted spread of a disease across a region can help in developing targeted prevention strategies.
- Animations: Animations offer a dynamic perspective on simulations, allowing us to see how variables change over time or in response to different inputs. This is especially useful for complex systems where multiple factors interact dynamically. For example, an animation showing the movement of air masses over a region could reveal potential patterns in weather patterns or climate change impacts.
Interpreting Simulation Results
Interpreting simulation outputs involves more than just visualization. It requires identifying key trends and patterns in the data to draw conclusions about potential future scenarios. Recognizing the limitations of the simulation model and considering potential uncertainties is paramount.
Uncertainty and Sensitivity Analysis
Acknowledging uncertainty is critical. Simulation results often involve inherent uncertainty due to the complexity of the system being modeled and the limitations of available data. Sensitivity analysis helps quantify how different inputs affect the outputs. This allows us to understand which factors have the greatest impact on the predicted outcomes and helps identify areas requiring further investigation.
For instance, a sensitivity analysis might show that changes in agricultural practices have a greater impact on food production than changes in irrigation methods, thus directing further research and model refinement efforts.
Presenting Simulation Results Effectively
Presenting simulation results clearly and concisely is essential. A well-structured presentation that communicates the key findings and caveats of the model is critical for stakeholders to make informed decisions. The presentation should clearly explain the methodology used, assumptions made, and limitations of the simulation. Transparent communication builds trust and ensures the results are used effectively.
Visualization Techniques Table
Visualization Technique | Description | Strengths | Weaknesses |
---|---|---|---|
Charts | Visual representation of data points using lines, bars, or other shapes to show trends and comparisons. | Easy to understand, effective for showing trends and comparisons. | Can be limited in representing complex relationships, may not be suitable for geographic data. |
Graphs | Visual representation of data points and their relationships, often using scatter plots, line graphs, or bar graphs. | Effective for identifying correlations and patterns between variables. | Can become complex for many variables, may not be suitable for large datasets. |
Maps | Visual representation of data points on a geographical map, often used to show spatial distributions. | Effective for visualizing geographically distributed data, providing spatial context. | May not be suitable for data without a clear geographical component. |
Animations | Dynamic visual representation of data changes over time, often showing how variables interact. | Provides a dynamic understanding of processes and relationships, useful for complex systems. | Can be more difficult to interpret than static visualizations, requires careful design to avoid confusion. |
Ethical Considerations and Societal Impacts
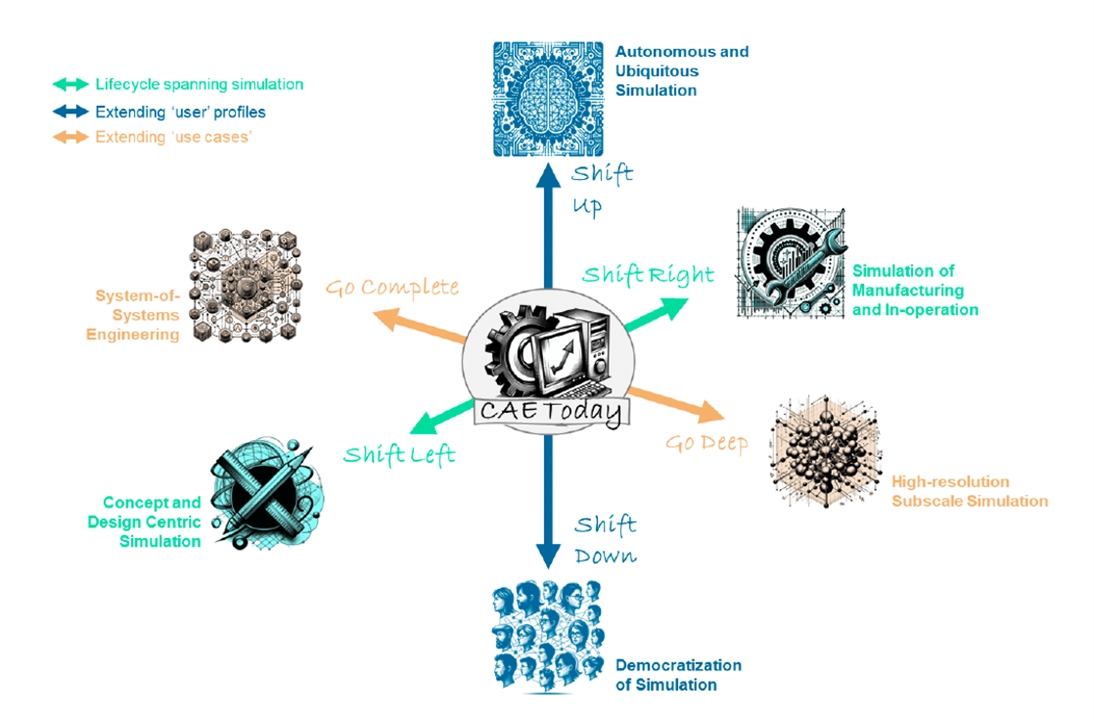
Future modeling with simulations, while powerful, presents significant ethical and societal challenges. The potential for bias in the data used to train these models, combined with the inherent uncertainty in predicting complex systems, requires careful consideration of the implications for different stakeholders. Misinterpretations of simulation outputs can lead to flawed policies and potentially harmful consequences.The use of simulations to predict future scenarios, whether for climate change, economic crises, or social trends, demands a nuanced approach that goes beyond technical accuracy.
It is crucial to acknowledge the limitations of these models and the potential for unintended consequences stemming from their application. The responsibility for utilizing these tools ethically and effectively falls on the shoulders of both model developers and the users of their output.
Ethical Implications of Using Simulations
Simulations, while offering valuable insights, can inadvertently perpetuate existing biases. These biases, stemming from the data used to train the models or from the assumptions embedded within the algorithms, can lead to skewed predictions that favor certain groups or perspectives. For example, if a model for predicting future crime rates is trained on data that disproportionately reflects crime in certain neighborhoods, it may exacerbate existing inequalities by reinforcing biased policing strategies.
Societal Impacts of Simulation Predictions
Misinterpretations of simulation outputs can have severe societal consequences. Policy decisions based on flawed predictions can lead to ineffective interventions or even exacerbate problems. For example, an economic model that inaccurately predicts the impact of a new trade policy could trigger significant job losses or economic instability. Moreover, the public perception of simulation outputs can influence public opinion and political discourse, potentially creating unnecessary anxieties or misdirected solutions.
Computer simulations are fascinating tools for predicting future scenarios, but the underlying hardware plays a crucial role. Intel’s recent focus on broadband wireless chips, like those detailed in this article ( intel to focus on broadband wireless chips ), could significantly impact the power and efficiency of these simulations, allowing for more complex models and faster processing. This means we can expect even more realistic and detailed projections in the future of computer simulations.
Potential Biases and Limitations
Computer simulations are only as good as the data they are trained on. If the data used to train the model contains biases or incomplete information, the resulting predictions will be flawed. Furthermore, simulations often struggle to capture the complexities of human behavior, social interactions, and unforeseen events. This inherent limitation means that simulations should not be seen as definitive truth but rather as valuable tools to explore possible future scenarios.
For instance, models predicting the spread of a disease may not accurately account for factors like social distancing or the development of new treatments.
Comparison of Ethical Frameworks
Different ethical frameworks offer diverse perspectives on the responsible use of simulations. Utilitarianism, for example, emphasizes maximizing overall societal well-being, prompting careful consideration of the potential benefits and harms of different simulation outputs. Deontology, conversely, focuses on upholding moral duties and principles, demanding that the development and use of simulations adhere to ethical standards. A virtue ethics approach would focus on the character and motivations of the individuals and institutions involved in the creation and application of these models.
Examples of Societal Challenge Addressing
Simulations have played a role in addressing various societal challenges. Climate change models have been used to predict future temperature increases and sea-level rise, enabling policymakers to implement mitigation strategies. Epidemiological models have aided in understanding disease transmission patterns and developing effective public health interventions. However, the application of simulations must always be accompanied by a critical evaluation of their limitations and potential biases.
These tools should be used as complements to, not replacements for, human judgment and ethical considerations.
Future Trends and Developments
The field of computer simulations is rapidly evolving, driven by advancements in computing power, algorithms, and data science. These developments are pushing the boundaries of what’s possible, allowing for increasingly complex and nuanced models of future scenarios. This dynamism creates opportunities to address intricate problems across diverse sectors, from climate change to healthcare.The future of simulation hinges on continuous innovation.
As computing capabilities improve, more intricate simulations can be constructed, offering deeper insights into the intricate workings of systems and processes. This allows for more accurate predictions and informed decision-making.
Emerging Trends in Simulation Technologies, Computer simulations modeling the future
The development of new simulation techniques and methodologies is reshaping the field. This includes the growing application of machine learning and artificial intelligence, which are proving powerful tools in enhancing simulation accuracy and efficiency. Techniques like agent-based modeling and coupled simulations are also gaining traction, allowing for more comprehensive and holistic analyses.
Advancements in Computing Power and Algorithms
Increased computational power allows simulations to handle larger datasets and more complex algorithms. This capacity is crucial for tackling intricate problems that were previously computationally intractable. For instance, climate models now incorporate more variables and geographic detail, producing more accurate predictions of future climate change. Sophisticated algorithms, such as those used in high-performance computing, are also vital for simulating intricate phenomena.
The Role of Artificial Intelligence in Enhancing Simulations
AI is revolutionizing simulation techniques. Machine learning algorithms can identify patterns and relationships within data, allowing for more accurate models and improved predictive capabilities. For example, in medical simulations, AI can analyze patient data to predict disease progression and personalize treatment strategies. Deep learning can enhance the realism of simulations by creating more sophisticated models of complex systems, such as traffic flow or financial markets.
Big Data and Enhanced Accuracy
Big data plays a significant role in improving the accuracy of future models. Access to massive datasets allows for more comprehensive analysis of historical trends and patterns, which in turn leads to more accurate predictions of future events. For instance, in urban planning, analyzing vast datasets of traffic patterns and population demographics helps urban planners design more efficient and resilient transportation systems.
Examples of Future Trends Shaping Future Modeling
The evolution of computer simulations is demonstrably influencing various sectors. For example, in the energy sector, simulations are used to design and optimize renewable energy infrastructure, predicting its impact on the environment and local communities. Similarly, in healthcare, simulations are employed to develop new drug therapies and treatment strategies.
End of Discussion
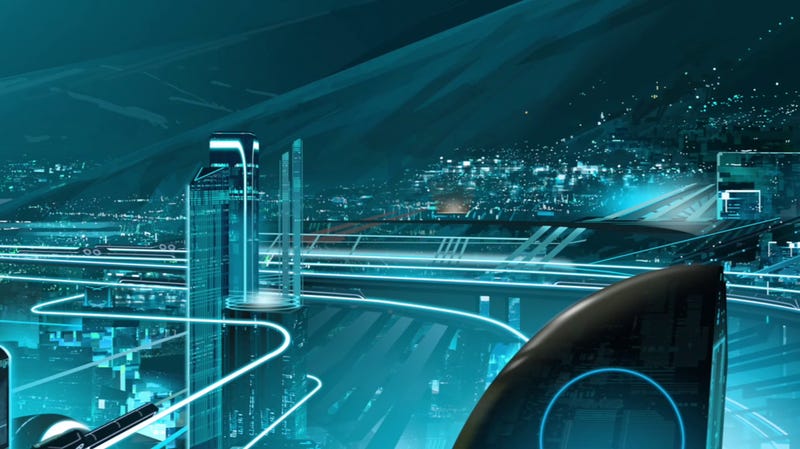
In conclusion, computer simulations modeling the future are proving to be invaluable tools for understanding complex systems and anticipating potential outcomes. Their applications span diverse fields, from economics to healthcare, offering insights into future trends. However, the ethical implications and limitations of these simulations need careful consideration. As technology advances, the potential for improved accuracy and broader application of these methods continues to grow, promising a more insightful and proactive approach to shaping our future.