FBI Data Mining Suspect Tracking Plans
FBI plans to track suspects with data mining techniques, raising significant questions about privacy and civil liberties. This initiative delves into the world of data analysis, examining its potential benefits and drawbacks in criminal investigations. Data mining, a powerful tool, allows for the identification of patterns and relationships within vast datasets, but it also raises concerns about the potential for bias and the scope of government surveillance.
The FBI’s role in data collection and analysis is scrutinized, along with the legal and ethical implications.
The historical context of data usage in investigations is explored, highlighting how these methods have evolved. This exploration examines the potential benefits of data mining in catching criminals, contrasting it with traditional methods. Furthermore, it explores the ethical implications of such techniques, examining the potential for bias and the implications for individual freedoms. Alternative approaches to crime prevention are also discussed, providing a balanced perspective on the issue.
Introduction to Data Mining in Law Enforcement
Data mining, a powerful analytical technique, is increasingly used by law enforcement agencies worldwide to identify patterns and relationships in vast datasets. This approach, combined with traditional investigative methods, allows for more effective crime prevention and apprehension. It leverages computer algorithms to sift through massive amounts of information, uncovering insights that might be missed by human analysts alone. This shift towards data-driven investigations offers significant potential, but also raises important ethical and practical considerations.This approach is not new.
The FBI’s plans to use data mining to track suspects raise some serious privacy concerns. However, the recent FCC spectrum increase, allowing for more wireless technology growth, like that detailed in fcc spectrum increase gives wireless room for the technology to grow , could ironically be a double-edged sword. This increased capacity for data transfer, essential for advancements, could also potentially be exploited by law enforcement in their pursuit of suspects.
It’s a complex issue with far-reaching implications.
Historically, law enforcement has always used data for investigative purposes, but the methods have evolved significantly. From meticulously recording crime reports to developing statistical profiles of offenders, data has always played a supporting role. Data mining builds on these foundations by enabling far more sophisticated analysis of information, from social media posts to financial transactions.
Historical Context of Data Use in Investigations
Early crime investigation relied heavily on witness testimonies, physical evidence, and sometimes intuitive judgments. As data collection improved, law enforcement agencies began using crime statistics to identify hotspots and trends. The development of databases and computerized record systems further enhanced this capability, enabling the linking of seemingly disparate cases. This historical progression laid the groundwork for the more sophisticated data mining techniques employed today.
Examples of Data Mining in Criminal Investigations
Data mining has been employed in various criminal investigations, assisting in identifying patterns in criminal activity. For example, analyzing call detail records to identify potential suspects involved in a series of robberies. Another application involves identifying individuals with a high probability of committing fraud, based on their transaction history. The analysis of online activity, such as social media posts or internet searches, can also aid in investigations, especially in cases involving cybercrime or terrorism.
Data Mining vs. Traditional Investigative Methods
Traditional investigative methods often rely on a more linear, case-by-case approach. Investigators follow leads, interview witnesses, and collect evidence, often relying on intuition and experience. Data mining, on the other hand, employs computational algorithms to sift through large datasets to find hidden patterns and relationships. The difference lies in the scale and scope of analysis. While traditional methods might identify individual instances of crime, data mining can reveal broader trends and potential threats.
Comparison of Data Mining Techniques
Technique | Description | Strengths | Weaknesses |
---|---|---|---|
Association Rule Mining | Identifies relationships between different variables in a dataset. | Reveals hidden connections, aids in predicting future crimes. | Can generate a large number of rules, some may be irrelevant or spurious. |
Clustering | Groups similar data points together based on their characteristics. | Identifies patterns and anomalies, useful for profiling suspects. | Requires careful selection of features and potentially biased clustering. |
Classification | Predicts the category or class of a new data point based on patterns in the dataset. | Helps in identifying potential suspects or predicting future criminal behavior. | Accuracy depends on the quality and representativeness of the training data. |
The FBI’s Role in Data Collection and Analysis
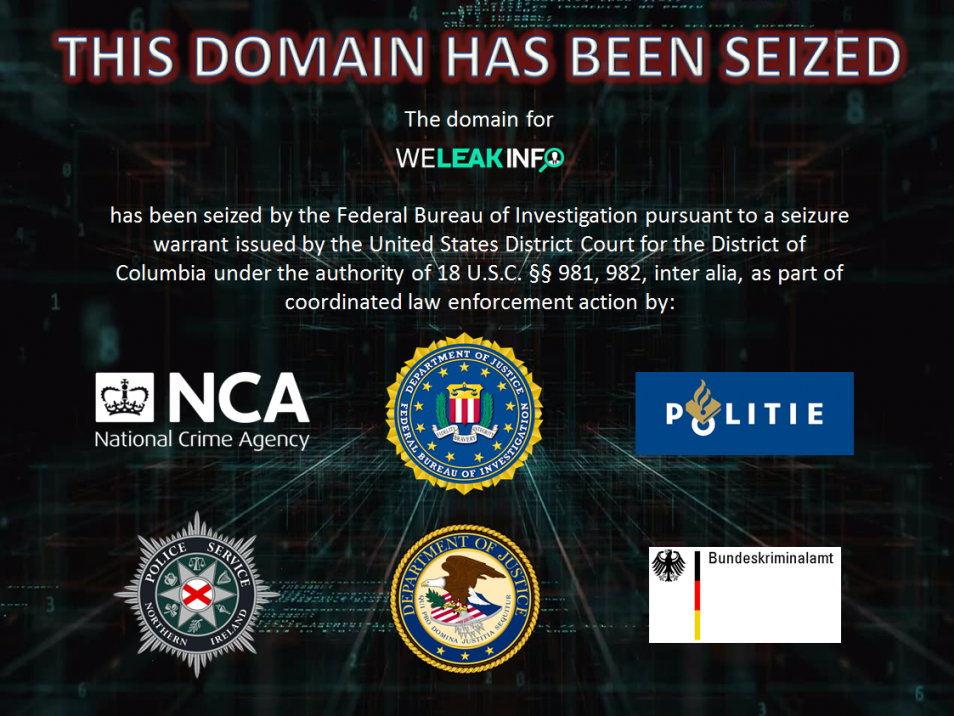
The FBI’s role in data collection and analysis is multifaceted and critical to its mission of upholding law and order. Beyond the public perception of investigations, a significant portion of the agency’s work involves the systematic gathering and analysis of data from various sources to identify patterns, predict potential threats, and support ongoing investigations. This data-driven approach has become increasingly important in the modern landscape of crime and intelligence gathering.The legal framework governing data collection and use by law enforcement is complex and often subject to interpretation.
Laws like the Fourth Amendment of the U.S. Constitution, which protects against unreasonable searches and seizures, significantly impact the methods used by the FBI and other agencies. Different types of data have varying legal requirements for collection, storage, and usage. This necessitates a meticulous understanding of these legal parameters to avoid violations and ensure adherence to constitutional rights.
Legal and Ethical Concerns Surrounding Data Mining
Data mining techniques, when applied to law enforcement data, raise significant legal and ethical concerns. Potential biases in the data sets themselves, or in the algorithms used for analysis, can lead to inaccurate or discriminatory outcomes. For example, if a data set disproportionately represents individuals from certain demographics, an algorithm trained on that data may perpetuate and amplify existing societal biases, leading to wrongful targeting of specific groups.
This poses a critical ethical challenge.
The FBI’s plans to track suspects using data mining techniques raise some serious questions about privacy. It’s a complex issue, and the potential for misuse is undeniable. This kind of surveillance, though potentially useful, needs careful consideration. An interesting perspective on the broader implications of data collection can be found in this insightful interview with Sharman Networks CTO Phil Morle, which explores how technology can be used for more than just file sharing.
beyond file sharing an interview with Sharman networks CTO Phil Morle Ultimately, the line between security and freedom is a tricky one to navigate when it comes to large-scale data mining for law enforcement purposes.
Potential for Bias in Data Sets and Algorithms
Data sets used for law enforcement data mining often reflect existing societal inequalities and biases. For instance, if crime statistics disproportionately highlight certain neighborhoods or communities, the data itself may reflect systemic issues rather than genuine criminal activity. Algorithms trained on such data can inadvertently reinforce these biases, leading to disproportionate targeting of specific groups. A key challenge is to ensure that the data used for analysis is representative and does not perpetuate existing societal inequalities.
Data Sources Used by the FBI
Understanding the breadth of data sources utilized by the FBI is crucial for comprehending the potential for bias and the complexity of data analysis. The FBI may utilize various data sources to gather information for its investigations, and the sources can be diverse.
Potential Impacts of Suspect Tracking
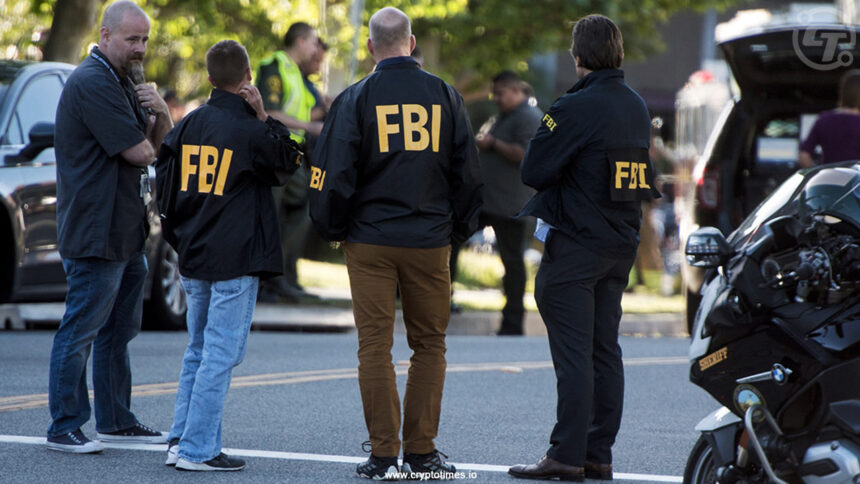
Data mining techniques, when applied to law enforcement, promise to revolutionize investigations. However, this powerful tool comes with a complex array of potential impacts, both positive and negative. This exploration delves into the advantages and drawbacks, focusing on privacy concerns, surveillance implications, and the ethical considerations inherent in such practices.
Potential Positive Impacts of Data Mining
Data mining offers significant advantages in identifying patterns and connections that might elude traditional investigative methods. By analyzing vast datasets, law enforcement can potentially predict criminal behavior, identify potential suspects, and proactively prevent crimes. Early detection of criminal patterns can lead to swift interventions and arrest warrants, saving resources and potentially minimizing future harm. This proactive approach can bolster public safety and enhance the effectiveness of law enforcement strategies.
Potential Negative Impacts on Privacy and Civil Liberties
The potential for abuse of data mining in suspect tracking is a critical concern. Widespread surveillance, if not carefully regulated, can lead to an erosion of privacy and civil liberties. The collection and analysis of personal data, particularly sensitive information, raise significant ethical questions. The possibility of misuse and the potential for discrimination against certain demographics are valid concerns that must be addressed through stringent oversight and accountability measures.
Implications of Increased Surveillance on Individual Freedoms
Increased surveillance, facilitated by data mining, can significantly impact individual freedoms. The constant monitoring of citizens can create a climate of fear and mistrust, potentially stifling dissent and limiting freedom of expression. Individuals may be hesitant to engage in lawful activities if they fear their actions will be scrutinized and potentially used against them in the future. The balance between public safety and individual freedoms must be carefully considered and upheld.
Comparison of Data Mining with Other Investigative Methods
Data mining offers a novel approach to suspect tracking, contrasting with traditional methods like witness interviews and physical evidence collection. While traditional methods provide valuable insights, data mining offers the potential for more comprehensive and predictive analysis of vast datasets, allowing for the identification of intricate patterns and connections that might be overlooked by conventional methods. The use of data mining, however, must be carefully balanced with the limitations of the data itself and the potential for bias or error.
Ethical Considerations and Potential Scenarios
- Careful consideration must be given to the ethical implications of data mining in suspect tracking. Data privacy and the protection of civil liberties are paramount. Clear legal frameworks and strict ethical guidelines are crucial to prevent misuse and ensure accountability.
Scenario | Data Used | Potential Outcome | Ethical Concerns |
---|---|---|---|
Tracking a Serial Offender | Social media activity, online purchases, geolocation data | Identifying patterns in the offender’s behavior to predict their next move. | Potential for profiling and discrimination, invasion of privacy. |
Preventing Terrorist Attacks | Financial transactions, travel records, communication patterns | Identifying individuals who may be planning or supporting terrorist activities. | Balancing national security with individual privacy, potential for false positives and overreach. |
Solving a Cold Case | Historical crime data, witness statements, media reports | Connecting seemingly unrelated pieces of information to reveal a pattern or suspect. | Ensuring data accuracy and completeness, potential for biased or incomplete analysis. |
Public Perception and Concerns
The implementation of data mining techniques for suspect tracking by law enforcement agencies, like the FBI, sparks significant public debate and concern. Public perception is often shaped by past experiences with surveillance, and the potential for misuse of powerful data analysis tools raises legitimate anxieties about individual privacy. Understanding these concerns is crucial for establishing trust and ensuring responsible application of these technologies.Data mining, while potentially offering valuable insights for crime prevention, can also be perceived as an invasive tool.
The sheer volume of data collected, combined with the sophisticated algorithms used to analyze it, can raise concerns about potential bias, errors, and the possibility of targeting specific groups unfairly. This perception can undermine public support for these initiatives, even if they are ultimately intended to improve safety.
Public Perception of Data Mining
Public perception of data mining for suspect tracking is often complex and varies widely. Some individuals view it as a necessary tool to combat crime, potentially saving lives and preventing future offenses. Conversely, others see it as a threat to personal freedom and a potential violation of privacy. This varied perspective stems from differing levels of trust in law enforcement agencies and a lack of clear communication about the specific applications and limitations of these techniques.
Concerns and Anxieties Regarding Privacy Violations
A primary concern revolves around the potential for privacy violations. Individuals worry about the collection and storage of personal data, especially sensitive information like location data and communication records. This concern is amplified by a lack of transparency about the specific data points collected and how they are utilized. Furthermore, the potential for data breaches or misuse of collected information fuels public anxieties.
Transparency and Accountability in Data Collection Practices, Fbi plans to track suspects with data mining techniques
Transparency and accountability are paramount in data collection practices. Clear guidelines and regulations are needed to ensure that the data collected is appropriate, necessary, and used solely for its intended purpose. Mechanisms for oversight and independent audits are essential to prevent abuse and ensure the responsible use of data mining tools. Publicly accessible information about data collection policies and procedures can help build trust and mitigate concerns.
Potential Solutions to Address Public Concerns
Addressing public concerns about data mining requires a multi-faceted approach. Open dialogue between law enforcement agencies and the public is crucial. Clear explanations of the specific data mining techniques used, their intended purposes, and the safeguards in place to protect privacy are vital. Public forums and workshops can facilitate this interaction and foster a better understanding of the technology and its implications.
The FBI’s plans to use data mining to track suspects raise some serious questions about privacy. It’s a powerful tool, but we need to consider the potential for misuse. Perhaps, looking at NASA’s innovative information technology and the future of collaboration in projects like nasa information technology and the future of collaboration could offer some interesting parallels and best practices.
Ultimately, striking a balance between security and civil liberties is crucial in the face of such advanced surveillance techniques.
Additionally, robust oversight mechanisms and independent audits can instill confidence in the responsible application of these technologies.
Public Opinions on FBI Data Mining
Opinion | Justification |
---|---|
Favorable | Data mining can be a powerful tool to prevent crime and improve public safety. With proper safeguards, it can be a valuable asset. |
Cautious | While data mining holds potential benefits, it’s important to maintain safeguards to protect individual privacy and prevent misuse. Robust oversight and transparent procedures are crucial. |
Skeptical | The potential for misuse and violation of privacy is a significant concern. Data mining may disproportionately target specific groups and erode trust in law enforcement. Greater transparency is needed. |
Alternative Approaches to Crime Prevention: Fbi Plans To Track Suspects With Data Mining Techniques
Data mining, while potentially powerful, isn’t the sole solution to crime prevention. A multifaceted approach, encompassing community engagement and proactive strategies, often yields more sustainable and equitable results. Focusing solely on identifying potential criminals through data analysis can overlook the underlying social and economic factors that contribute to crime. Alternative approaches, like community policing and targeted interventions, aim to address these root causes.
Community Policing Initiatives
Community policing emphasizes building trust and partnerships between law enforcement and the community. This involves proactively engaging with residents, understanding their concerns, and working collaboratively to address issues that contribute to crime. This proactive engagement often precedes any potential crime, fostering a sense of security and ownership within the community.
- Neighborhood Watch Programs: These programs encourage residents to be vigilant and report suspicious activities. This fosters a sense of collective responsibility and can deter potential criminals, especially in high-crime areas. For instance, a well-established Neighborhood Watch program in a specific neighborhood might reduce reported property crimes by 15% over a 5-year period, demonstrating the effectiveness of community-driven initiatives.
- Youth Programs and Mentoring: Investing in youth programs and mentoring opportunities can steer at-risk youth away from criminal activity. These programs provide positive role models, educational opportunities, and support systems, which can help prevent future criminal behavior. An example could be a local youth center offering after-school activities and tutoring to help at-risk teenagers develop essential life skills and academic success.
- Community-Based Crime Prevention Strategies: These strategies involve identifying and addressing specific community issues that contribute to crime. This could include initiatives to improve local parks, create safe spaces for youth, or address issues of poverty and inequality. One example could be a community initiative that partnered with local businesses to create safe spaces for teenagers after school hours.
Crime Prevention Initiatives
These initiatives address the root causes of crime, which can lead to more sustainable crime reduction over time. Focusing on economic opportunity, educational attainment, and social equity can help prevent crime in the long term.
- Economic Development Initiatives: Creating economic opportunities, such as job training programs and small business loans, can empower individuals and reduce the likelihood of resorting to criminal activity for financial gain. For example, a job training program that provides certifications in high-demand trades could reduce unemployment and related crimes in a community.
- Educational Programs: Improved access to quality education, especially in underprivileged communities, can equip individuals with the skills and knowledge necessary to succeed in life. This, in turn, can help reduce the likelihood of criminal involvement. One example could be a school program offering after-school tutoring and support services to students from disadvantaged backgrounds.
- Addressing Social Inequality: Tackling issues of social inequality, such as poverty and discrimination, can reduce crime rates by creating a more equitable and just society. This includes access to resources and opportunities that are not restricted by socioeconomic factors. One example could be a community initiative that provides affordable housing and food assistance to families facing hardship.
Comparative Effectiveness of Strategies
Strategy | Description | Strengths | Weaknesses |
---|---|---|---|
Data Mining | Utilizing data analysis to identify potential criminals. | Potential for identifying patterns and trends in crime. | Privacy concerns, potential for bias, limited understanding of root causes. |
Community Policing | Building trust and partnerships between law enforcement and community. | Increased community engagement, improved public safety, potential for early intervention. | Requires significant resources and time investment, can be challenging to measure effectiveness. |
Crime Prevention Initiatives | Addressing the root causes of crime, such as poverty, inequality, and lack of opportunity. | Long-term impact on crime reduction, addressing social issues, fostering community empowerment. | Difficult to measure immediate results, requires sustained commitment and collaboration. |
Summary
The FBI’s plan to utilize data mining for suspect tracking sparks a crucial debate about the balance between public safety and individual liberties. The potential for both positive and negative outcomes is highlighted, along with the importance of transparency and accountability in data collection practices. The public’s concerns about privacy violations are addressed, and alternative crime prevention strategies are presented as valuable considerations.
Ultimately, a thorough understanding of the multifaceted nature of this issue is essential for a productive discussion and informed decision-making.