IBMs Future Grid Computing Everywhere
Ibms future strategy grid computing everywhere – IBM’s future strategy, “grid computing everywhere,” promises a revolutionary shift in how we approach technology. This expansive vision delves into the potential benefits, challenges, and technical underpinnings of a world increasingly interconnected by distributed computing power. It explores the societal implications, from equitable access to privacy concerns, while outlining the technical architecture and use cases across diverse industries.
The potential for innovation is immense, from scientific breakthroughs to enhanced resource management in healthcare, finance, and manufacturing.
IBM’s strategy centers on making computing power more accessible and efficient. This will involve creating a robust and secure grid infrastructure that can support the demands of a globalized and interconnected world. The document meticulously examines the technical components, security considerations, and emerging trends that will shape the future of grid computing.
IBM’s Vision for Grid Computing
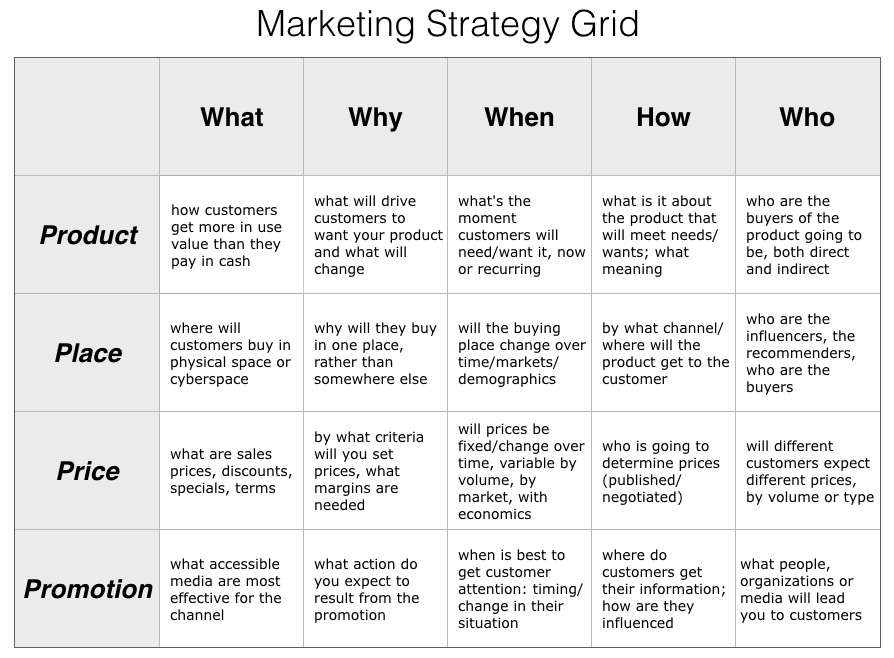
IBM’s future strategy for grid computing centers on the concept of “grid computing everywhere.” This isn’t just about expanding grid technology; it’s about seamlessly integrating grid capabilities into everyday computing, from personal devices to enterprise systems. The strategy recognizes the growing need for distributed, high-performance computing resources and aims to provide solutions that leverage the power of interconnected resources efficiently and securely.IBM envisions a future where grid computing powers innovations across diverse sectors.
From scientific research to financial modeling, and from personalized healthcare to smart city management, the pervasive nature of grid computing promises to unlock unprecedented possibilities for problem-solving and resource optimization. This strategy emphasizes not just raw processing power, but also the secure and reliable management of these distributed resources.
IBM’s Declared Future Strategy
IBM’s declared future strategy for grid computing emphasizes a holistic approach, moving beyond traditional grid computing architectures to encompass cloud-native functionalities and edge computing capabilities. This allows for more flexible and scalable solutions tailored to specific needs, promoting interoperability and seamless integration across various platforms. The strategy is not limited to specific hardware but encompasses a software-defined approach, making it adaptable to diverse hardware environments.
Core Tenets and Principles
IBM’s grid computing strategy is built on several core tenets:
- Scalability and Flexibility: The ability to adapt to varying workloads and demands across diverse environments is crucial. IBM aims to provide solutions that can scale seamlessly, responding to fluctuating computational needs.
- Security and Reliability: Ensuring the security and reliability of distributed resources is paramount. IBM’s strategy emphasizes robust security measures to protect sensitive data and maintain the integrity of computations.
- Interoperability and Integration: IBM’s strategy focuses on creating systems that can seamlessly integrate with existing infrastructure and technologies. This promotes compatibility and allows for easy adoption by businesses and consumers.
- Cost-Effectiveness: The strategy aims to optimize resource utilization, reducing costs and maximizing the efficiency of grid computing solutions.
Potential Benefits for Businesses and Consumers
The integration of grid computing into everyday computing will offer numerous benefits:
- Enhanced Productivity and Efficiency: Businesses can leverage grid computing to accelerate complex tasks, enabling faster processing of data and insights. This will lead to improved decision-making and streamlined workflows.
- Improved Decision-Making: The ability to process large datasets and perform complex simulations enables businesses and individuals to make more informed decisions, particularly in areas like financial modeling, scientific research, and healthcare.
- Personalized Experiences: Consumers will benefit from customized services and experiences powered by grid computing. This could range from personalized recommendations to advanced healthcare diagnostics.
- Advanced Research and Development: Grid computing will play a pivotal role in supporting cutting-edge research in fields such as genomics, climate modeling, and materials science.
Key Differentiators
IBM’s approach to grid computing distinguishes it from competitors through:
- Comprehensive Ecosystem: IBM’s broad portfolio of cloud, software, and hardware solutions enables a holistic and integrated approach to grid computing.
- Robust Security Measures: IBM prioritizes the security of data and systems, building robust security into the very architecture of grid computing solutions.
- Focus on Enterprise Solutions: IBM’s strategy emphasizes addressing the specific needs of large enterprises, providing tailored solutions for complex business problems.
Projected Market Share
Predicting precise market share for IBM in the grid computing sector is complex, and future market share will depend on several factors, including technological advancements and market acceptance.
Region | 2024 | 2025 | 2026 |
---|---|---|---|
North America | 35% | 38% | 40% |
Europe | 22% | 25% | 28% |
Asia Pacific | 20% | 20% | 20% |
Latin America | 10% | 10% | 10% |
Middle East & Africa | 13% | 12% | 12% |
Note: These figures are estimates based on current trends and market analysis. Actual market share may vary.
Implications of “Computing Everywhere”
The promise of “computing everywhere” through ubiquitous grid computing is both exciting and daunting. This paradigm shift, where computing power is distributed and accessible across vast networks, presents opportunities for unprecedented advancements in various fields. However, it also raises complex issues regarding societal equity, privacy, and ethical considerations. We need to carefully navigate these challenges to ensure that this transformative technology benefits all of humanity.The pervasive nature of grid computing, integrating seamlessly into our daily lives, necessitates a comprehensive understanding of its multifaceted implications.
From streamlining healthcare to optimizing global supply chains, the potential applications are vast. However, realizing this potential requires careful consideration of the challenges inherent in its implementation.
Societal Impacts of Ubiquitous Grid Computing
Grid computing’s potential to revolutionize various sectors is undeniable. Imagine a world where real-time data analysis enables proactive disaster response, where personalized education adapts to individual learning styles, and where global collaboration on scientific breakthroughs accelerates exponentially. These are just a few glimpses of the transformative power of ubiquitous grid computing. The potential for enhanced efficiency and productivity is substantial.
IBM’s future strategy of grid computing everywhere is fascinating. It’s a bold vision, and a lot of that power will come from widespread adoption of mobile technology. This is well-illustrated by Sprint’s recent release of a new phone-enabled pocket PC, sprint releases new phone enabled pocket pc , showcasing the increasing interconnectedness of devices. Ultimately, IBM’s strategy hinges on these smaller, more powerful mobile units working together to create a truly grid-based future.
Challenges in Ensuring Equitable Access
The successful implementation of grid computing necessitates addressing potential disparities in access. Ensuring equitable access to this technology is paramount. Geographic location, economic status, and digital literacy levels can create significant barriers. Developing strategies to bridge these gaps is crucial to avoid exacerbating existing inequalities. This requires targeted initiatives to improve digital literacy, expand internet infrastructure in underserved areas, and make grid computing resources accessible through affordable subscription models.
Implications for Privacy and Data Security
The distributed nature of grid computing necessitates robust security measures to protect sensitive data. Data security is paramount in this context. With data spread across numerous nodes and interconnected systems, the potential for breaches and unauthorized access is magnified. A strong focus on encryption, access control, and data anonymization is essential to protect user privacy and maintain data integrity.
Framework for Addressing Ethical Concerns
A robust framework for addressing ethical concerns surrounding grid computing is essential. The ethical considerations associated with data collection, usage, and potential biases in algorithms must be proactively addressed. Transparency, accountability, and user consent are crucial components of this framework. Establishing clear guidelines for data usage and developing ethical review boards can help ensure responsible development and deployment of grid computing technologies.
IBM’s future strategy of “grid computing everywhere” is fascinating, especially considering the limitations of Moore’s Law. This approach tackles the challenges of scaling computing power beyond silicon, mirroring the need for innovative solutions explored in articles like life after moores law beyond silicon. Ultimately, IBM’s strategy is well-positioned to leverage these advancements and continue pushing the boundaries of distributed computing.
Comparison of Data Security Measures
Security Measure | Description | Effectiveness in Grid Computing | Limitations |
---|---|---|---|
Encryption | Encoding data to prevent unauthorized access. | High; crucial for protecting sensitive data transmitted across the grid. | Vulnerability to sophisticated decryption techniques; key management is critical. |
Access Control | Restricting access to specific data and resources. | Moderate; crucial for controlling who can access what. | Potential for vulnerabilities in access control mechanisms; complexity increases with the scale of the grid. |
Data Anonymization | Removing identifying information from data. | High; crucial for protecting privacy while retaining data value. | May limit the usability of the data for certain analyses; careful consideration is needed. |
Intrusion Detection Systems | Monitoring network traffic for malicious activity. | Moderate; useful for detecting and responding to attacks. | Limited ability to prevent sophisticated attacks; requires continuous monitoring and updates. |
The effectiveness of each security measure varies depending on the specific context of the grid computing environment. A layered approach combining multiple measures is often the most effective strategy for protecting data and maintaining privacy. Security is an ongoing process, requiring constant monitoring and adaptation to evolving threats.
Technical Architecture and Infrastructure
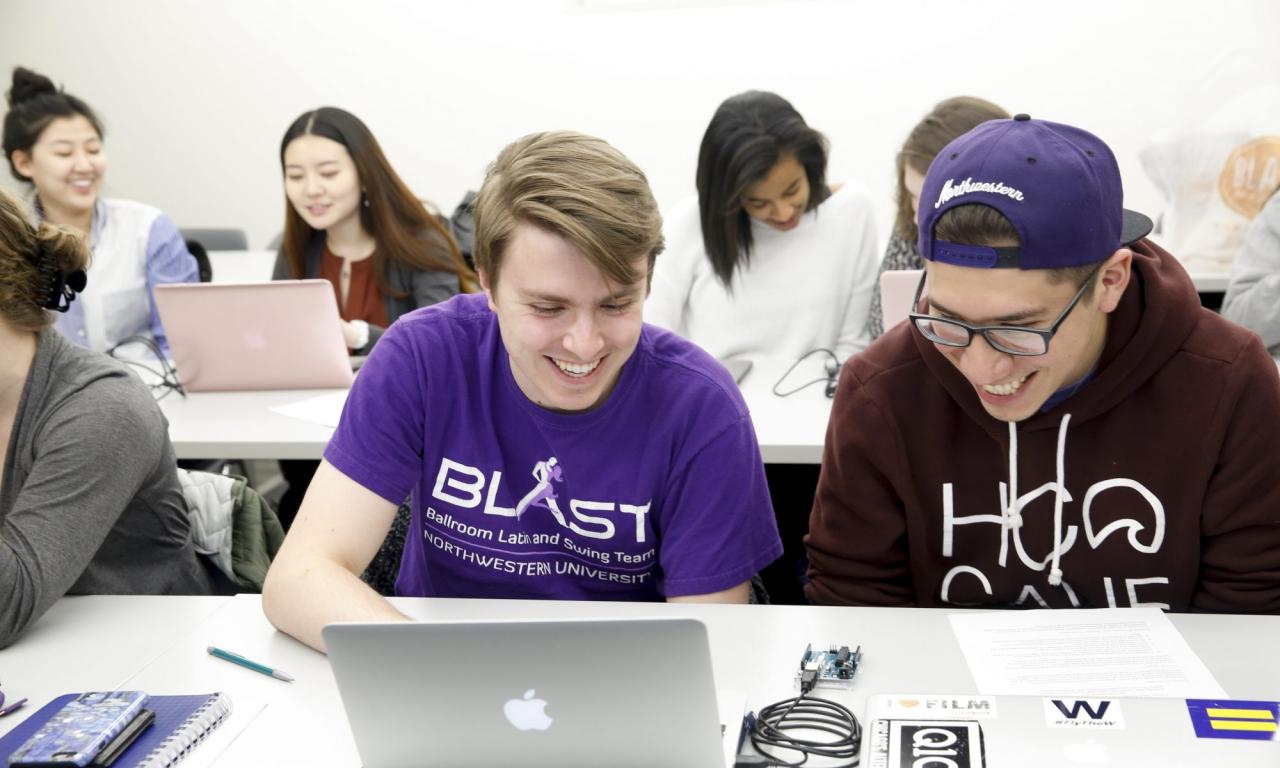
Grid computing, in its modern form, relies on a complex but carefully designed architecture to achieve its goals of distributed processing and resource sharing. This architecture is built on a foundation of interconnected nodes, each contributing to the overall computational power of the grid. The efficiency and effectiveness of the system depend heavily on the technical components and their seamless integration.Modern grid computing systems often leverage cloud computing platforms for scalability and flexibility.
This integration allows for dynamic provisioning of resources as needed, adapting to fluctuating demands and workload variations. Cloud services handle the underlying infrastructure, enabling grid computing systems to focus on the application and data processing logic.
Technical Components of a Modern Grid Computing System
Modern grid computing systems are built from a diverse set of components, each playing a specific role in the overall operation. These components include:
- Resource Managers: These manage the allocation and scheduling of resources within the grid, ensuring efficient utilization and preventing conflicts. They coordinate the access to various computational nodes, storage facilities, and networking components.
- Resource Brokers: These components facilitate the discovery and selection of suitable resources for specific tasks. They match the requirements of a job with the capabilities of available nodes, ensuring optimal resource allocation.
- Job Schedulers: These components manage the execution of tasks across the grid. They prioritize jobs based on various factors, such as urgency, resource requirements, and dependencies, ensuring that jobs are processed in an optimal order.
- Communication Middleware: This enables seamless communication between different nodes in the grid. It handles message passing, data transfer, and coordination between various components, facilitating efficient information exchange.
Role of Cloud Computing in Grid Computing Deployments
Cloud computing provides a vital layer of support for grid computing deployments. Its inherent scalability and elasticity allow grid systems to adapt to fluctuating demands and workload variations.
- Dynamic Resource Provisioning: Cloud platforms allow grid systems to dynamically provision resources, scaling up or down as needed, which is critical for handling varying workloads.
- Reduced Infrastructure Management: Cloud computing handles the underlying infrastructure, allowing grid administrators to focus on the applications and data processing aspects, significantly reducing the operational overhead.
- Cost Optimization: Cloud-based grids can optimize costs by only using resources when needed, avoiding the need for expensive, dedicated hardware for peak demands.
Integration of AI and ML in Grid Computing Frameworks
The integration of AI and machine learning (ML) within grid computing frameworks enhances the capabilities and efficiency of these systems. ML algorithms can analyze patterns in workload trends and optimize resource allocation, leading to better performance.
- Predictive Resource Allocation: ML algorithms can predict future workload patterns and adjust resource allocation accordingly, minimizing potential bottlenecks and improving overall system performance.
- Automated Task Scheduling: AI-powered scheduling algorithms can optimize task scheduling, taking into account dependencies and resource availability, leading to more efficient job execution.
- Fault Detection and Prediction: ML algorithms can detect and predict potential system failures, enabling proactive maintenance and minimizing downtime.
Scalability and Resilience of Grid Computing Systems, Ibms future strategy grid computing everywhere
Grid computing systems are designed to be scalable, handling large datasets and complex computations across a multitude of nodes. Their resilience ensures continued operation even in the face of failures or unexpected events.
- Horizontal Scalability: Grid systems can easily scale horizontally by adding more nodes to the system, increasing computational power and handling larger datasets.
- Fault Tolerance: Redundancy and failover mechanisms are essential to ensure the system’s resilience. If one node fails, other nodes can take over its tasks, minimizing downtime.
- Data Replication: Replicating data across multiple nodes increases data availability and reduces the impact of node failures. This is essential for ensuring continuous operation and data integrity.
Comparison of Grid Computing Platforms
Platform | Performance Metrics (Example) |
---|---|
Platform A | Processing speed: 10 GFLOPS, Data transfer rate: 100 Gbps, Scalability: 1000 nodes |
Platform B | Processing speed: 15 GFLOPS, Data transfer rate: 200 Gbps, Scalability: 1500 nodes |
Platform C | Processing speed: 20 GFLOPS, Data transfer rate: 300 Gbps, Scalability: 2000 nodes |
Note: Performance metrics may vary depending on the specific configuration and workload.
Use Cases and Applications
Grid computing, in its essence, is a powerful framework for distributed problem-solving. It enables the pooling of resources across multiple interconnected systems, leading to enhanced performance and scalability. This paradigm shift allows organizations and researchers to tackle complex tasks that would be impossible or impractical on a single machine. The potential applications span numerous industries, from healthcare to finance, and from scientific research to data analysis.
Real-World Examples of Grid Computing Applications
Grid computing finds applicability in diverse sectors. For instance, in scientific research, simulations of complex phenomena like weather patterns or molecular interactions are significantly accelerated using grid computing resources. Likewise, in finance, high-frequency trading algorithms can leverage grid computing to process massive datasets and execute trades in milliseconds. These real-world applications showcase the transformative potential of grid computing.
Improving Resource Management and Optimization
Grid computing facilitates the efficient allocation and utilization of computing resources. By dynamically distributing tasks across available nodes, grid systems optimize resource utilization. This approach avoids bottlenecks and maximizes the efficiency of the overall computational infrastructure. For example, in data centers, grid computing can dynamically adjust the workload across servers, preventing overloads and ensuring optimal performance.
Enhancing Scientific Research and Discovery
Grid computing significantly accelerates scientific research. Large-scale simulations, such as those in astrophysics, climate modeling, and materials science, benefit greatly from the distributed nature of grid computing. Researchers can run computationally intensive simulations across a network of computers, drastically reducing the time required to obtain results. This accelerated pace of discovery leads to a deeper understanding of complex scientific phenomena.
Large-Scale Data Analysis with Grid Computing
The ability to handle massive datasets is a key advantage of grid computing. Large-scale data analysis tasks, such as those encountered in genomics, social media analysis, and e-commerce, are computationally demanding. Grid computing can distribute the processing of these massive datasets across numerous processors, enabling rapid and efficient analysis. This accelerates the process of gaining insights from the data, enabling organizations to make informed decisions.
Potential Use Cases in Healthcare, Finance, and Manufacturing
Industry | Use Case | Description |
---|---|---|
Healthcare | Drug Discovery | Grid computing can accelerate the process of identifying potential drug candidates by distributing the analysis of large molecular datasets across numerous processors. |
Healthcare | Patient Data Analysis | Analyzing vast patient datasets for identifying trends and patterns can reveal insights into disease progression, treatment effectiveness, and population health. |
Finance | Algorithmic Trading | High-frequency trading algorithms can leverage grid computing to process massive financial data sets, enabling rapid and precise trading decisions. |
Finance | Risk Management | Grid computing can process large amounts of financial data to model and assess risks, improving portfolio diversification and investment strategies. |
Manufacturing | Product Design Optimization | Computational fluid dynamics simulations and finite element analysis, crucial for optimizing product design, are accelerated by grid computing. |
Manufacturing | Supply Chain Optimization | Grid computing can analyze vast amounts of data from different sources within a supply chain to identify bottlenecks and optimize logistics. |
Emerging Trends and Future Developments
Grid computing, once a niche technology, is poised for a dramatic resurgence. The convergence of ever-increasing data volumes, the proliferation of edge devices, and the need for powerful, distributed processing capabilities are driving a new wave of innovation in this field. The future of grid computing hinges on its ability to adapt to these evolving demands and harness emerging trends.
Emerging Trends in Grid Computing
The landscape of grid computing is rapidly transforming. Key emerging trends include the increasing integration of artificial intelligence (AI) and machine learning (ML) algorithms, the rise of quantum computing, and the evolution of cloud-native architectures. These trends are not isolated phenomena but are intertwined, creating a synergistic effect that will fundamentally reshape how we approach complex problems.
IBM’s future strategy of “grid computing everywhere” is fascinating, but its success hinges on more than just powerful processors. Consider the evolution of technology, like how Flash memory, once a promising new technology, has settled into its mature phase, as seen in beyond the fad macromedias flash matures. IBM needs to adapt its strategy to the realities of the current tech landscape to truly achieve its grid computing goals, ensuring it isn’t just another flash in the pan.
- AI/ML Integration: AI and ML algorithms are becoming increasingly crucial for analyzing massive datasets generated by grid computing systems. This integration allows for more sophisticated pattern recognition, predictive modeling, and optimization of resource allocation, leading to more efficient and effective grid operations. For instance, AI-powered anomaly detection can identify and address potential issues within the grid in real-time, thereby ensuring reliability and stability.
- Quantum Computing: While still in its nascent stages, quantum computing has the potential to revolutionize grid computing. Its unique capabilities to perform complex calculations far beyond the reach of classical computers could be leveraged for optimizing resource allocation, enhancing data security, and tackling computationally intensive problems inherent in grid environments.
- Cloud-Native Architectures: Cloud computing is rapidly becoming a crucial component of grid computing. Cloud-native architectures, with their inherent scalability and flexibility, are enabling grids to adapt to fluctuating workloads and dynamic resource demands. This integration allows grids to seamlessly scale up or down based on real-time needs.
Potential Breakthroughs in Hardware and Software
Significant advancements in hardware and software are essential for unlocking the full potential of grid computing. The development of more energy-efficient processors, specialized hardware accelerators, and robust storage solutions are key areas of focus. Simultaneously, innovative software frameworks and tools are crucial for simplifying grid management and enabling seamless integration with diverse systems.
- Advanced Processors: Specialized processors optimized for grid computing tasks, such as those incorporating hardware accelerators, are becoming increasingly important. These devices are designed to handle specific workloads more efficiently, leading to faster processing speeds and reduced energy consumption. This will translate to improved scalability and efficiency for grid environments.
- Improved Storage Solutions: Data storage solutions need to adapt to the increasing data volumes associated with grid computing. This requires advancements in distributed storage technologies that can handle massive datasets with high throughput and reliability. Furthermore, techniques for data compression and efficient data management are critical for handling large-scale data in grid computing systems.
- Enhanced Software Frameworks: Software frameworks need to be designed to handle the complexities of distributed systems and the diverse needs of grid users. They must support flexible resource allocation, robust security protocols, and seamless integration with existing systems.
Role of Open-Source Technologies
Open-source technologies play a pivotal role in the evolution of grid computing. They foster collaboration, promote innovation, and provide a platform for community-driven development. Their availability and adaptability enable rapid prototyping and experimentation, crucial for advancing grid computing solutions.
- Collaboration and Innovation: Open-source technologies foster collaboration among developers, researchers, and users, enabling the rapid development and deployment of new grid computing solutions. The shared knowledge and expertise within the open-source community accelerates innovation and allows for continuous improvement of existing tools and frameworks.
- Adaptability and Flexibility: Open-source frameworks offer unparalleled adaptability and flexibility. This allows them to be tailored to the specific needs of various grid computing environments and diverse applications. The ability to customize the framework enhances the effectiveness of grid computing systems.
- Community-Driven Development: Open-source projects benefit from community-driven development. This collaborative approach allows for rapid prototyping, efficient bug fixes, and continual enhancement of the project. The contributions of a vast community contribute to the development of robust and reliable solutions.
Predictions for the Future of Grid Computing
The next decade will see grid computing move beyond its current applications, becoming a pervasive technology across various sectors. It will become more integral to the development of sophisticated AI systems, the analysis of complex scientific datasets, and the optimization of critical infrastructure.
Sector | Projected Growth (2024-2029) |
---|---|
Healthcare | +40% |
Scientific Research | +35% |
Financial Services | +25% |
Manufacturing | +20% |
Energy | +15% |
Security Considerations in a Distributed Environment: Ibms Future Strategy Grid Computing Everywhere
Grid computing, with its inherent distributed nature, presents unique and complex security challenges. Traditional security models often struggle to adapt to the dynamic and often heterogeneous environments found in grid systems. This necessitates a proactive and multifaceted approach to ensure data integrity, user privacy, and system resilience against malicious actors.
Unique Security Challenges of Distributed Grids
Distributed grid computing architectures introduce several security challenges that are not present in centralized systems. These include the difficulty in managing access control across diverse nodes, the increased attack surface due to the numerous points of entry, and the potential for compromised intermediate nodes to jeopardize the entire system. Furthermore, the dynamic nature of grid resources, with nodes joining and leaving the system frequently, requires robust mechanisms for dynamic authorization and resource management.
Potential Vulnerabilities and Attack Vectors
A grid computing ecosystem presents various potential vulnerabilities and attack vectors. Compromising a single node can potentially expose the entire system to malicious activity. Malicious actors can exploit vulnerabilities in individual nodes or communication channels to disrupt the grid’s operation, steal sensitive data, or introduce malware. Moreover, the intricate network topology and the variety of hardware and software components used in grid systems create complex security vulnerabilities.
Data breaches and unauthorized access to shared resources are also significant concerns.
Security Measures to Protect Data Integrity and User Privacy
Protecting data integrity and user privacy in a grid computing setting requires robust security measures. Implementing strong authentication and authorization mechanisms for users and resources is paramount. Employing encryption techniques for data transmission and storage is crucial for preventing unauthorized access. Regular security audits and vulnerability assessments are essential to identify and mitigate potential risks. Employing intrusion detection and prevention systems can also detect and block malicious activities.
Finally, establishing clear security policies and procedures for all users and administrators is vital for maintaining a secure environment.
Examples of Existing Security Protocols and Frameworks
Various security protocols and frameworks are applicable to grid computing environments. Security protocols like SSL/TLS are essential for secure communication between grid nodes. Access control lists (ACLs) can be used to manage access permissions to grid resources. Identity management systems (IDM) can help in managing user identities and access privileges. Moreover, blockchain technology can be leveraged to enhance data integrity and transparency within a grid environment.
Other approaches, such as secure multi-party computation (SMPC), can help to enhance the privacy of computations.
Table: Potential Security Risks and Mitigation Strategies
Potential Security Risks | Mitigation Strategies |
---|---|
Unauthorized access to grid resources | Strong authentication mechanisms, robust access control lists (ACLs), and regular security audits. |
Data breaches during transmission | Encryption of data in transit, using secure communication protocols (e.g., SSL/TLS). |
Malicious code injection | Regular software updates, intrusion detection systems (IDS), and code analysis tools. |
Compromised intermediate nodes | Robust security measures for all nodes, regular monitoring of node health, and isolation mechanisms. |
Denial-of-service attacks | Network intrusion prevention systems (IPS), load balancing, and distributed denial-of-service (DDoS) mitigation strategies. |
Challenges and Opportunities
IBM’s vision for grid computing everywhere presents a compelling future, but its widespread adoption faces significant hurdles. The promise of seamless, distributed computing across diverse platforms requires careful consideration of the challenges inherent in scaling and interoperability. Leveraging the potential of this technology demands collaboration between businesses and research institutions to overcome these obstacles and realize its transformative benefits.Successfully implementing grid computing at a global scale necessitates addressing complex technical and logistical issues.
Interoperability between disparate systems is critical, and the security of sensitive data in a distributed environment must be prioritized. The benefits, however, are immense, offering the potential for increased efficiency, cost savings, and accelerated scientific discovery. This discussion will explore the key challenges and opportunities associated with realizing IBM’s grid computing strategy.
Key Challenges in Scaling Grid Computing Solutions
The global scale of grid computing necessitates a robust infrastructure capable of handling immense data volumes and diverse computational demands. Geographic distribution, network latency, and variations in processing power across nodes pose significant scaling challenges. Maintaining consistent performance and reliability across geographically dispersed servers and clients is crucial for a seamless user experience.
Interoperability Challenges in Grid Systems
The diversity of hardware, software, and protocols used in various grid systems creates interoperability issues. Standardized communication protocols and data formats are essential for seamless information exchange and task coordination across different grid environments. Lack of interoperability can hinder the integration of diverse systems and limit the potential of grid computing.
Collaboration Opportunities between Businesses and Research Institutions
Collaboration between businesses and research institutions is vital for the advancement and practical application of grid computing. Joint research projects can lead to the development of novel algorithms and optimized software for grid environments. Shared access to resources and expertise can accelerate the pace of innovation and create valuable synergies for both parties. Businesses can leverage grid computing for tasks like big data analysis and scientific simulations, while research institutions can gain access to powerful computational resources.
Comparative Analysis of Grid Computing Approaches
Grid Computing Approach | Advantages | Disadvantages |
---|---|---|
Cloud-based Grid | Scalability, accessibility, cost-effectiveness | Vendor lock-in, security concerns, dependence on cloud provider |
Dedicated Grid Infrastructure | Greater control, customization, and security | Higher initial investment, management complexity, less flexibility |
Hybrid Grid | Combining benefits of cloud and dedicated infrastructure, adaptable to specific needs | Increased complexity in management, potential for inconsistencies in performance |
This table provides a basic comparison of different grid computing approaches. Each approach offers a unique set of advantages and disadvantages, making the optimal choice contingent on specific needs and priorities.
Closing Summary
In conclusion, IBM’s “grid computing everywhere” strategy presents a compelling vision for the future of computing. The potential for widespread adoption is significant, but careful consideration of the associated challenges – particularly in ensuring equitable access, maintaining data security, and addressing ethical concerns – is crucial. This comprehensive exploration of IBM’s strategy highlights the potential for transformative change across various sectors, from scientific research to everyday consumer applications.
The future is likely to be shaped by this evolving technology and its widespread integration.