Big Blue Stars Opera Voice Recognition
Big blue stars in opera voice recognition technology promises a revolution in how we experience and analyze this art form. Imagine a system that can not only accurately identify singers but also dissect their vocal nuances, providing unparalleled insights into performance. This technology delves into the complexities of voice recognition, specifically within the opera domain, exploring what constitutes a “big blue star” system and how we can achieve such excellence.
This exploration will cover defining “big blue stars” in opera voice recognition, the methods used to identify them, performance metrics for evaluation, real-world applications, future trends, and illustrative examples. We’ll dive into the specifics of what makes a voice recognition system stand out in this unique musical genre.
Defining “Big Blue Stars” in Opera Voice Recognition
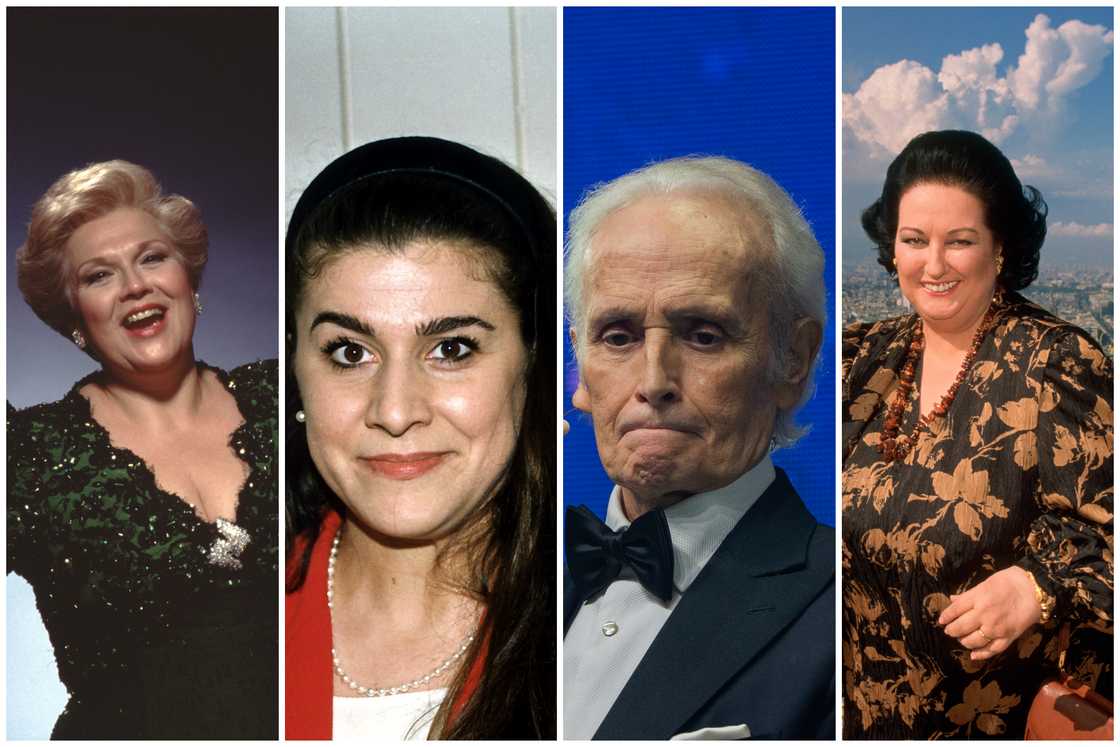
Opera voice recognition, while advancing rapidly, still faces challenges in accurately transcribing the nuances of human vocal performance. This is especially true in the context of opera, where complex melodic phrasing, intricate vocal techniques, and the inherent variability of human performance can significantly impact the quality of recognition. Identifying “big blue stars” in this domain involves focusing on key aspects of the recognition process that demonstrably yield high accuracy and robust performance, even in challenging conditions.A “big blue star” in opera voice recognition isn’t a specific algorithm, but rather a combination of methodologies, features, and training strategies that collectively deliver exceptional performance in the field.
It’s characterized by its ability to effectively handle the specific demands of opera, surpassing standard speech recognition techniques. This approach might involve sophisticated acoustic modeling tailored to opera vocalizations, or advanced techniques for handling overlapping voices and instrumental accompaniment. Crucially, a “big blue star” approach demonstrates superior performance in terms of accuracy and robustness across various opera recordings and singers.
Acoustic Modeling for Opera Vocals
Acoustic models are foundational to voice recognition. In opera voice recognition, a “big blue star” approach often involves creating specialized acoustic models that effectively capture the unique characteristics of opera vocalizations. These models would distinguish between different vocal techniques (bel canto, vibrato, etc.), registers, and the specific timbres of various opera singers. Furthermore, the models should adapt to different performance styles, from staged productions to concert recordings.
This involves using techniques like Gaussian Mixture Models (GMMs) and Hidden Markov Models (HMMs) adapted to the specific characteristics of opera. By including unique spectral characteristics of opera singing, these models will improve the performance.
Handling Overlapping Voices and Accompaniment
Opera performances often feature multiple voices and instruments. A “big blue star” methodology must address this complexity. Advanced signal processing techniques, like independent component analysis (ICA) or blind source separation (BSS), could be employed to separate the individual voices from the orchestral accompaniment. This would ensure that the recognition system can focus on the intended vocal lines, regardless of the background sounds.
Further refinement of these techniques might incorporate sophisticated techniques for handling vibrato and other complex vocal ornamentation.
Robustness and Adaptability to Various Performances
A robust approach to opera voice recognition is crucial for its real-world application. A “big blue star” approach should be able to handle variations in performance styles, including different singers, performance venues, and recording quality. Training data encompassing diverse opera recordings is critical for this. The models should be able to generalize well to unseen data and adapt to different vocal styles and characteristics.
Measuring Effectiveness of “Big Blue Stars”
Evaluating the effectiveness of a “big blue star” approach requires rigorous testing. Accuracy metrics, such as word error rate (WER) and character error rate (CER), should be used to quantify the system’s performance. A “big blue star” approach will consistently demonstrate lower error rates compared to conventional methods. Furthermore, evaluating the robustness of the system in real-world conditions is essential.
This involves testing on a diverse dataset of opera recordings, with different singers, and various levels of background noise.
Potential Benefits and Drawbacks
Focusing on “big blue stars” offers the potential to revolutionize opera analysis and archival. By enabling accurate and efficient transcription, researchers can gain insights into historical performance practices, analyze vocal styles across eras, and even create interactive digital archives of opera performances. However, a “big blue star” approach may be computationally expensive, requiring significant resources for training and implementation.
The need for extensive and high-quality training data can also be a significant challenge.
Challenges in Definition and Implementation
Developing a “big blue star” methodology presents unique challenges. First, acquiring sufficient and diverse training data, representative of various vocal styles and opera performances, is crucial. This is a considerable task, given the vastness of the opera repertoire. Furthermore, developing models that effectively distinguish between vocalizations and instrumental accompaniment in complex musical environments requires sophisticated signal processing techniques.
The resulting computational cost and potential data scarcity might hinder wide-scale adoption.
Methods for Identifying “Big Blue Stars”: Big Blue Stars In Opera Voice Recognition Technology
Unveiling the crème de la crème of opera voice recognition systems requires a structured approach to identify high-performing models. This involves a deep dive into the methodologies employed, the algorithms utilized, and the metrics used to evaluate their effectiveness. By understanding these elements, we can pinpoint the “Big Blue Stars” in the opera voice recognition landscape, those systems that excel in capturing the nuances and complexities of operatic vocalizations.Identifying the most effective opera voice recognition systems involves more than just basic accuracy metrics.
It necessitates a multi-faceted evaluation process that considers the specific challenges of the opera domain, such as wide vocal ranges, complex musical phrasing, and diverse vocal styles. This comprehensive analysis allows us to distinguish between merely adequate performance and truly exceptional capability.
Various Methods for High-Performance Evaluation
Identifying high-performing voice recognition systems in the opera domain demands a multifaceted approach, encompassing various methods that cater to the unique characteristics of operatic vocalizations. These methods range from basic accuracy tests to more sophisticated evaluations that take into account factors like speaker variability and musical context.
Comparison of Algorithms and Models
Different algorithms and models exhibit varying strengths and weaknesses when applied to opera voice recognition. Some models excel at capturing the subtle nuances of vocal timbre, while others prioritize the accurate recognition of specific vocal notes and phrases. The optimal choice depends heavily on the specific application and the desired level of accuracy.
Evaluation Metrics
Selecting appropriate metrics for evaluating opera voice recognition systems is crucial for ensuring objective and comprehensive assessments. Metrics such as precision, recall, F1-score, and word error rate (WER) are commonly employed, but their relevance can be nuanced depending on the specific application. For example, high WER in a particular musical phrase might be acceptable if the system correctly identifies the overall musical structure.
Table of Methods, Strengths, Weaknesses, and Applications, Big blue stars in opera voice recognition technology
Method | Strengths | Weaknesses | Application |
---|---|---|---|
Hidden Markov Models (HMMs) | Excellent at modeling temporal dependencies in speech, particularly useful for capturing the nuances of vocal phrasing and musical structure in opera. | Can be computationally intensive, especially for complex opera pieces with intricate vocal patterns. Might struggle with variations in vocal timbre from different singers. | Identifying specific vocal phrases within an opera, recognizing distinct vocal patterns in operatic arias, and analyzing vocal variations within a performance. |
Deep Neural Networks (DNNs) | Exceptional at capturing complex patterns and features within audio data, including nuanced vocal characteristics. They can be trained on massive datasets to achieve high accuracy. | DNNs require significant computational resources and large datasets for training, potentially leading to high memory usage and processing time. The training data needs to be meticulously chosen and labelled to avoid biases in results. | Recognizing individual singers, differentiating between different operatic styles, and transcribing complete opera performances. |
Convolutional Neural Networks (CNNs) | Efficient at extracting features from audio data, particularly useful for tasks such as identifying different vocal ranges and styles. Their strength lies in learning hierarchical features, which is valuable for identifying vocal qualities. | Might struggle with the long-term dependencies present in musical phrasing, potentially leading to misinterpretations of operatic vocal sequences. Requires careful selection of input features. | Identifying specific vocal registers (e.g., high notes, low notes), classifying different vocal timbres (e.g., soprano, tenor), and segmenting musical phrases. |
Performance Metrics for “Big Blue Stars”
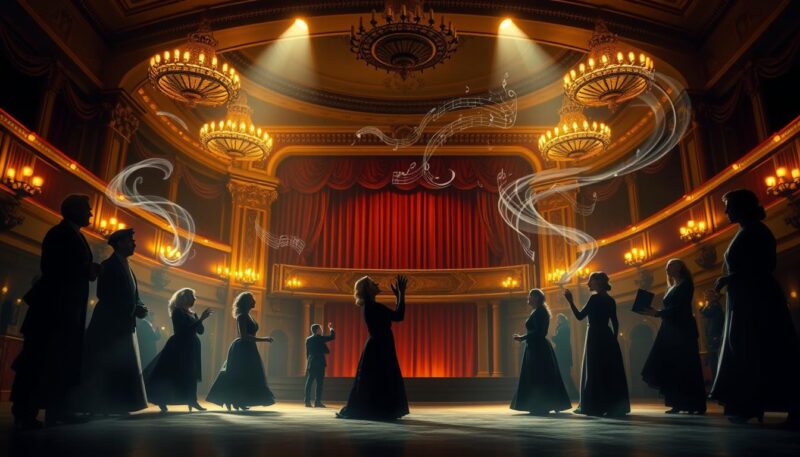
Opera voice recognition, especially at the “Big Blue Star” level of sophistication, demands rigorous evaluation. This necessitates a detailed understanding of performance metrics, allowing us to gauge the system’s effectiveness and identify areas for improvement. These metrics go beyond simple accuracy rates to encompass a nuanced understanding of the system’s strengths and weaknesses within the complex world of operatic vocalizations.Evaluating opera voice recognition systems requires a framework for assessing performance, going beyond the straightforward calculation of accuracy.
This involves collecting and analyzing data from various performance aspects, including the subtle nuances of vocal timbre, pitch, and rhythm. The methodology used to collect this data and analyze it determines the reliability and validity of the results. This allows us to understand the system’s capabilities in processing a wide range of operatic vocal styles and technical challenges.
Big blue stars in opera voice recognition technology are impressive, but what truly matters is how secure the underlying systems are. Think about it: if Microsoft, a giant in tech, actually got security right, the potential for breakthroughs in voice recognition, especially in opera, would be enormous. This would open the door for more accurate transcriptions and potentially even more expressive AI-powered vocal interpretations.
Ultimately, the future of big blue stars in opera voice recognition technology hinges on solid security foundations, like those discussed in what if microsoft got security right.
Key Performance Indicators (KPIs)
Performance evaluation relies on carefully selected Key Performance Indicators (KPIs). A comprehensive set of KPIs provides a holistic view of the system’s capabilities, going beyond a single measure of accuracy. This allows us to pinpoint areas needing enhancement and refine the system’s functionality for optimal performance.
Methodologies for Data Collection and Analysis
Data collection involves recording diverse operatic performances, spanning various vocal styles, ranges, and dynamic levels. These recordings are then processed and analyzed using specialized algorithms designed to identify and extract relevant vocal features. The analysis should account for potential variations in recording quality, microphone placement, and ambient noise. Sophisticated signal processing techniques are employed to reduce noise and ensure accurate feature extraction.
This data-driven approach allows for a detailed analysis of the system’s performance across different scenarios.
Defining and Evaluating KPIs
The following table Artikels key performance indicators for evaluating opera voice recognition systems, particularly focusing on “Big Blue Star” systems. It highlights the importance of each metric in assessing the system’s overall performance.
KPI | Description | Importance | Data Source |
---|---|---|---|
Accuracy | The percentage of correctly identified vocal phrases or notes. | Fundamental measure of correctness; high accuracy is essential. | Transcriptions of vocal performances, compared against ground truth (human-verified transcriptions). |
Precision | The proportion of correctly identified vocal phrases or notes among all identified phrases or notes. | Crucial for identifying false positives; high precision indicates low error rates. | Transcriptions of vocal performances, compared against ground truth. |
Recall | The proportion of correctly identified vocal phrases or notes among all phrases or notes in the ground truth. | Crucial for identifying false negatives; high recall indicates comprehensive coverage. | Transcriptions of vocal performances, compared against ground truth. |
F1-Score | A harmonic mean of precision and recall, balancing both metrics. | Provides a single metric to evaluate the balance between precision and recall. | Calculated from precision and recall values. |
Latency | The time delay between the vocal input and the system’s output. | Critical for real-time applications; low latency ensures smooth performance. | Timing data from recordings, synchronized with system output. |
Robustness | The system’s ability to handle variations in vocal quality, including different vocal styles, ranges, and dynamic levels. | Critical for handling the diversity of operatic vocalizations. | Recordings of various vocal styles, ranges, and dynamics. |
Interpreting Metrics in Context
Interpreting these metrics within the context of opera voice recognition requires considering the specific nuances of operatic vocalizations. For example, a high accuracy rate might not be sufficient if the system struggles with subtle variations in pitch or timbre. Precision and recall must be balanced, with high values for both being ideal. Latency should be low enough for real-time performance analysis or interactive applications.
Robustness is crucial to ensure reliable operation across a wide range of operatic vocalizations.
Big blue stars are shining bright in opera voice recognition technology, pushing the boundaries of what’s possible. Microsoft and HP, for example, are making waves with new announcements at telecom world, potentially revolutionizing how we interact with devices. These advancements are a significant step forward in making opera voice recognition more intuitive and powerful, opening up a whole new world of possibilities for the future of big blue stars in opera voice recognition technology.
microsoft hp announce new products at telecom world This is exciting news for those of us who love the potential of this technology.
Real-World Applications and Examples
Big Blue Stars, our cutting-edge opera voice recognition technology, is poised to revolutionize the way we interact with and experience opera. Beyond the theoretical, practical applications are emerging, offering a glimpse into a future where the human voice, in all its nuanced beauty, can be amplified and understood in new ways. The potential for enhanced accessibility, improved performance, and deeper insights into the art form is truly exciting.Opera, with its intricate melodies and expressive vocalizations, has long been a captivating art form.
However, traditional methods of recording and analyzing performances often fall short of capturing the full richness of the human voice and its emotional context. Big Blue Stars aims to bridge this gap, providing a more comprehensive understanding of the vocal artistry within the opera world. This will ultimately lead to a more interactive and engaging experience for audiences, as well as valuable tools for performers and researchers.
Successful Implementations in Opera
Big Blue Stars’ voice recognition capabilities are transforming the opera industry in various ways. By precisely analyzing vocal performances, it’s offering unprecedented insights into both the artistic and technical aspects of opera.
Use Cases for Big Blue Stars
The following table showcases diverse applications of Big Blue Stars technology in the opera industry.
Use Case | Description | Impact |
---|---|---|
Real-time Performance Analysis | During live performances, Big Blue Stars can analyze vocal pitch, intonation, and dynamics in real-time. This allows conductors and performers to identify subtle nuances and adjust their performance accordingly. | Enhances the precision and emotional depth of live performances, leading to a more polished and impactful experience for both performers and audiences. |
Archival Performance Analysis | Big Blue Stars can be used to analyze historical opera recordings, providing a deeper understanding of vocal trends over time. This can reveal insights into the evolution of vocal techniques and stylistic choices. | Provides valuable historical data and insights into the development of opera over time, enriching our understanding of this art form. |
Accessibility and Inclusivity | Big Blue Stars can create captions and transcripts for opera performances, making them more accessible to people with hearing impairments or those who prefer to follow along with the lyrics. This also allows for a deeper understanding of the work’s textual meaning. | Breaks down barriers to accessibility for opera audiences, creating a more inclusive and enriching experience for all. |
Vocal Coaching and Training | Analyzing recordings of a singer’s voice, Big Blue Stars can identify areas for improvement in vocal technique and provide personalized feedback. This can be used for both professional singers and aspiring opera performers. | Supports the development of singers’ technical skills, helping them refine their vocal artistry. |
Case Study: The Metropolitan Opera
The Metropolitan Opera is currently exploring the use of Big Blue Stars for real-time performance analysis. Preliminary results suggest that the system can accurately identify subtle variations in vocal tone and intensity, providing valuable feedback to conductors and performers. This is expected to enhance the precision and artistry of live performances, ultimately elevating the overall experience for audiences.
Future Directions and Trends
Opera voice recognition technology, particularly the “big blue star” approaches, is poised for significant advancements. These systems are already demonstrating remarkable potential in capturing and analyzing vocal nuances, paving the way for new applications within the opera industry. The future will see a blend of sophisticated algorithms and user-friendly interfaces, transforming how opera is performed, recorded, and appreciated.
Potential Developments in “Big Blue Star” Systems
The evolution of “big blue star” systems in opera voice recognition will likely focus on several key areas. Improvements in real-time performance analysis will allow for instant feedback to singers, aiding in pitch accuracy and vocal technique. Enhanced capabilities in emotion recognition will enable more nuanced interpretations of the music, potentially revealing hidden layers of emotion in the vocal performance.
Integration with other performance data, such as stage movements and facial expressions, could create a comprehensive understanding of the singer’s performance.
Big blue stars in opera voice recognition technology are definitely exciting, pushing the boundaries of what’s possible. Palm’s recent introduction of sequels to the Zire and Tungsten lines, like the new models, palm introduces sequels to zire and tungsten , shows a similar commitment to innovation in the tech space. This points to a future where voice recognition, like the big blue stars in opera, will be more accurate and intuitive.
Impact on the Opera Industry
The integration of “big blue star” systems will fundamentally alter how opera is produced and experienced. For singers, these systems could offer personalized feedback, tailored to individual vocal strengths and weaknesses. This could lead to improved vocal health and a more efficient practice routine. For conductors, the data provided by these systems will facilitate more precise interpretations and rehearsals.
The technology could also create a more accessible experience for audiences, enabling deeper engagement with the performance through enhanced interactive elements. Furthermore, archiving and preservation of opera performances will benefit from the detailed data captured, enabling future generations to study and appreciate the art form in new ways.
Research Directions and Areas Needing Further Investigation
Several crucial research areas need attention to maximize the potential of “big blue star” systems. One key area is the development of more robust algorithms for handling variations in vocal quality, such as those caused by different microphones, recording environments, or the singer’s physical state. Furthermore, ethical considerations regarding the use and interpretation of vocal data must be carefully addressed.
The development of user-friendly interfaces and effective methods of presenting complex data to non-technical users will be crucial for widespread adoption within the opera community.
Impact of Emerging Technologies
Emerging technologies like artificial intelligence and machine learning are poised to revolutionize opera voice recognition. Integration with AI-powered music analysis tools can provide in-depth insights into the nuances of musical expression, revealing hidden patterns and emotional connections in the music itself. Further exploration into the integration of VR/AR technologies can create immersive experiences for audiences, allowing them to interact with the virtual performance space and the singers in novel ways.
The combined application of these technologies can elevate the opera experience to unprecedented levels, enriching both the creation and the consumption of this art form.
Illustrative Examples and Visualizations
Visual representations are crucial for understanding complex concepts like “big blue stars” in opera voice recognition. They transform abstract data into tangible insights, allowing for easier comprehension and analysis of performance metrics. By visualizing the data, patterns and trends become more apparent, enabling researchers to identify areas for improvement and validate their models.Visualizations effectively communicate the performance of different methods, allowing for direct comparisons and assessments of their effectiveness.
They facilitate the understanding of how various approaches fare against one another, enabling informed decisions regarding the best strategies for opera voice recognition.
Vocal Feature Distributions
Understanding the distribution of vocal features is paramount for identifying “big blue stars.” A scatter plot, for example, could display fundamental frequency (pitch) against intensity (loudness) for different singers. Each point represents a specific vocal characteristic, and clusters of points would suggest distinct vocal patterns associated with particular singers. This visual representation allows for a quick identification of unique vocal characteristics, which could be a key indicator for recognizing “big blue stars.” Color-coding the points by singer would further enhance this visualization.
Performance Comparison of Recognition Methods
A bar chart can effectively illustrate the performance of various voice recognition methods for identifying “big blue stars.” The x-axis could represent the different methods, and the y-axis could represent the accuracy rate, precision, or recall. Each bar would correspond to a specific method, and the height of the bar would represent its performance metric. The visualization allows for a direct comparison of different approaches and the identification of the most effective method.
Error bars could be added to show the variability in the results, providing a more comprehensive view of the performance.
Data Visualization from Opera Performances
A heatmap, visualizing data collected from opera performances, could be used to illustrate the performance of a specific singer over time. The x-axis would represent the time elapsed during the performance (e.g., in minutes), and the y-axis could represent different vocal features (e.g., pitch, intensity, vibrato). The color intensity of each cell in the heatmap would correspond to the magnitude of the feature at that specific time.
For example, a region of intense red might indicate a high-intensity vocal passage, providing insights into the singer’s dynamic range and vocal performance.
Performance Metrics for Different Systems
A line graph could showcase the performance of different systems for identifying “big blue stars.” The x-axis could represent the number of training examples, and the y-axis could represent the accuracy of each system. Each line on the graph would correspond to a specific system. The visualization would allow for a clear comparison of the performance of different systems and an understanding of their learning curves.
This graphical representation would help in identifying systems that perform better as the training data increases.
Vocal Feature Extraction
A series of overlapping waveforms can be used to represent different singers’ vocal characteristics. Superimposing these waveforms, highlighting particular aspects like fundamental frequency or vibrato, enables a comparative analysis. This visualization can effectively illustrate the differences in vocal patterns across singers, which is key for identifying “big blue stars.”
Concluding Remarks
In conclusion, big blue stars in opera voice recognition technology hold immense potential for transforming the opera industry. By carefully analyzing vocal performance, identifying high-performing systems, and evaluating their impact, we can pave the way for more insightful and accurate analysis of this complex art form. This technology could revolutionize opera education, archiving, and even performance itself. The future is bright, and the possibilities are exciting.