Neural Network Technology Moves Mainstream
Neural network technology moves into the mainstream, ushering in a new era of technological advancement. From the early days of research to today’s widespread applications, this journey has been remarkable. This blog post delves into the factors driving this mainstream adoption, exploring the key applications, technological advancements, and the evolving public perception. We’ll also examine the challenges and future trends shaping this transformative technology.
This post explores how neural networks are becoming more integrated into our daily lives, from the mundane to the revolutionary. We’ll see how they are impacting various industries, from healthcare to finance, and the potential societal impact. The evolution of neural networks from niche technology to ubiquitous tool is a fascinating story of innovation, adoption, and societal change.
Introduction to Mainstream Adoption
Neural networks, a subset of artificial intelligence, are rapidly transforming various sectors. These complex systems, inspired by the human brain, learn from data to identify patterns, make predictions, and perform tasks that once required explicit programming. Their ability to automate complex processes and extract valuable insights from vast datasets has led to their widespread adoption across industries.The historical context of neural networks reveals a fascinating journey.
Early neural network models, while promising, faced limitations in computational power and data availability. This led to periods of both excitement and disillusionment. However, advances in computing power, coupled with the exponential growth of data, have revitalized the field. This renewed interest has resulted in significant breakthroughs and the development of increasingly sophisticated algorithms.Several factors have propelled neural networks into the mainstream.
The availability of massive datasets, particularly in image recognition, natural language processing, and other fields, provides the raw material for training these models. The development of powerful GPUs (Graphics Processing Units) has significantly accelerated the training process, enabling the handling of complex tasks previously considered computationally intractable. Finally, advancements in algorithms and architectures have made neural networks more efficient and accurate.
Open-source frameworks and libraries have also played a crucial role in democratizing access to these powerful tools.
Early Adoption vs. Current Mainstream Adoption
A comparison of early neural network adoption with the current mainstream adoption reveals a stark contrast. The initial enthusiasm was met with limited applications, mostly in academic research. The current mainstream adoption, on the other hand, is characterized by widespread practical application across diverse industries.
Feature | Early Adoption (1980s-1990s) | Current Mainstream Adoption (2010s-present) |
---|---|---|
Time | Limited computational resources, scarce data availability. | Exponential growth of data, powerful GPUs, efficient algorithms. |
Applications | Limited to academic research, specific niche applications (e.g., handwritten digit recognition). | Image recognition (e.g., self-driving cars, medical diagnosis), natural language processing (e.g., chatbots, language translation), finance (e.g., fraud detection), and more. |
Impact | Limited impact beyond research circles. | Significant impact across multiple sectors, driving innovation and automation. |
Public Awareness | Minimal, largely confined to specialized communities. | Increased awareness, with mainstream media coverage highlighting advancements and applications. |
Applications and Impact

Neural networks are no longer a futuristic concept; they are rapidly transforming industries and shaping our society. From image recognition in smartphones to sophisticated financial modeling, the practical applications are diverse and impactful. This widespread adoption necessitates careful consideration of both the benefits and potential pitfalls.
Key Applications of Neural Networks
Neural networks are demonstrating exceptional performance across a range of applications. Their ability to learn complex patterns from vast datasets allows them to automate tasks previously requiring human expertise. This leads to increased efficiency and accuracy in many areas.
- Image Recognition and Object Detection: Neural networks are crucial for image recognition in various fields, from medical diagnosis to self-driving cars. Deep learning models can identify objects, people, and patterns in images with remarkable accuracy, surpassing human capabilities in some cases. This is driving advancements in fields like autonomous vehicles, where real-time object detection is critical for safe navigation.
- Natural Language Processing: Neural networks power many natural language processing tasks, including machine translation, sentiment analysis, and chatbots. They enable computers to understand and generate human language, leading to more intuitive and efficient communication between humans and machines. Examples include virtual assistants, language translation apps, and sophisticated customer service chatbots.
- Financial Modeling and Prediction: Neural networks are used to model complex financial markets and predict future trends. Their ability to identify patterns in vast datasets allows for more accurate risk assessments and investment strategies. This can lead to significant improvements in portfolio management and risk mitigation in financial institutions.
Impact on Industries, Neural network technology moves into the mainstream
The impact of neural networks extends far beyond specific applications. They are reshaping entire industries by automating tasks, improving decision-making, and creating new possibilities.
- Healthcare: Neural networks are revolutionizing healthcare through applications like medical image analysis, drug discovery, and personalized medicine. Early detection of diseases, more accurate diagnoses, and the development of targeted therapies are becoming increasingly common. For instance, neural networks can analyze medical images to detect anomalies, like cancerous tumors, with greater precision and speed than human radiologists.
- Manufacturing: Neural networks are used for predictive maintenance, quality control, and optimizing production processes in manufacturing. By analyzing sensor data, neural networks can anticipate equipment failures and optimize resource allocation, leading to increased efficiency and reduced downtime. This allows manufacturers to reduce costs and improve productivity.
- Retail: Neural networks are transforming the retail experience through personalized recommendations, inventory management, and fraud detection. Understanding customer preferences and predicting demand allows for more efficient stock management and targeted marketing campaigns.
Societal Impact
The widespread use of neural networks will undoubtedly impact society in profound ways. Automation driven by these networks will reshape the job market, requiring workforce adaptation and potentially leading to job displacement in certain sectors. This requires proactive measures to support retraining and upskilling initiatives.
Ethical Considerations
As neural networks become more integrated into society, ethical considerations become paramount. Bias in data can lead to biased outcomes in neural networks, requiring careful attention to data collection and training methods. Transparency and explainability of decisions made by neural networks are crucial for building trust and ensuring fairness. Accountability and responsibility for the actions of automated systems are also important issues to address.
Sectors Impacted by Neural Networks
Sector | Application | Impact |
---|---|---|
Healthcare | Medical image analysis, drug discovery, personalized medicine | Improved diagnosis accuracy, faster treatment, and development of targeted therapies. |
Finance | Fraud detection, risk assessment, algorithmic trading | Enhanced security, more accurate risk management, and potentially higher returns on investment. |
Manufacturing | Predictive maintenance, quality control, process optimization | Reduced downtime, increased efficiency, and lower operational costs. |
Retail | Personalized recommendations, inventory management, customer service | Improved customer experience, enhanced efficiency, and increased sales. |
Transportation | Self-driving cars, traffic optimization | Increased safety, reduced congestion, and potential for improved transportation efficiency. |
Technological Advancements
The mainstream adoption of neural network technology is inextricably linked to significant advancements across hardware, algorithms, and software. These innovations have dramatically lowered the barriers to entry, making neural networks accessible to a wider range of researchers, developers, and businesses. This has fueled a surge in applications and, consequently, a broader impact on various sectors.
Hardware Advancements: GPUs
The rise of powerful graphics processing units (GPUs) has been instrumental in enabling faster and more efficient neural network training. GPUs excel at parallel processing, a crucial aspect of neural network computations. Their ability to handle massive datasets and complex calculations at high speeds has significantly reduced training times. This acceleration has been pivotal in pushing neural networks beyond research labs and into practical applications.
For example, companies like Nvidia have developed specialized hardware tailored for deep learning, further accelerating the process and fostering innovation.
Algorithm and Software Advancements
Significant progress in neural network algorithms and software libraries has streamlined the development process. These advancements have allowed researchers and developers to focus more on the application and less on the technical complexities of implementation. Libraries like TensorFlow and PyTorch provide user-friendly interfaces for building and training neural networks, simplifying the process and making it more accessible to a wider range of users.
The development of more sophisticated algorithms, such as convolutional neural networks (CNNs) for image recognition and recurrent neural networks (RNNs) for natural language processing, has also led to substantial improvements in performance. Furthermore, advancements in optimization techniques have led to more efficient training and better model performance.
Different Types of Neural Networks
Various types of neural networks cater to different needs and tasks. Convolutional Neural Networks (CNNs) excel at image recognition and processing, playing a crucial role in applications like object detection and image classification. Recurrent Neural Networks (RNNs) are particularly well-suited for sequential data, enabling applications in natural language processing, time series analysis, and speech recognition. Feedforward neural networks remain fundamental, forming the building blocks for many more complex architectures.
Each type of network has specific strengths and weaknesses, leading to their specialized use cases within the broader field of artificial intelligence.
Summary of Key Technological Innovations
Innovation | Description | Impact on Neural Network Adoption |
---|---|---|
Powerful GPUs | High parallel processing capabilities, optimized for deep learning tasks. | Dramatically reduced training times, enabling large-scale training and experimentation. |
Sophisticated Algorithms (CNNs, RNNs) | Specific algorithms tailored for image recognition, natural language processing, and other tasks. | Led to significant performance improvements and enabled new applications in diverse domains. |
User-friendly Software Libraries (TensorFlow, PyTorch) | Simplified the development process for building and training neural networks. | Increased accessibility to neural networks, attracting a broader developer base. |
Public Perception and Understanding
The mainstream adoption of neural networks brings with it a need for the public to understand these powerful technologies. Public perception significantly influences the acceptance and responsible development of neural networks. A clear and accurate understanding of their capabilities, limitations, and potential impacts is crucial for informed public discourse and policy-making.Public understanding of neural networks is not uniform.
While some grasp the fundamental concepts and applications, others remain largely unaware or harbor misconceptions, often fueled by media portrayals. This makes it essential to analyze how the public narrative evolves, how the media presents neural networks, and the level of public awareness.
Media Portrayals of Neural Networks
Media portrayals of neural networks are varied, sometimes highlighting their potential benefits and other times focusing on potential risks. News articles, documentaries, and even fictional narratives shape the public’s understanding.
- Positive portrayals frequently focus on applications like medical diagnosis, self-driving cars, and personalized learning, highlighting their efficiency and potential to improve lives. These stories often emphasize the technological marvel and advancement.
- Negative portrayals sometimes focus on the potential for bias, job displacement, or misuse in areas like surveillance or autonomous weapons systems. These narratives often highlight the potential for harm or unintended consequences.
Evolution of the Public Narrative
The public narrative surrounding neural networks has evolved from a largely unknown technology to one with both enthusiastic support and cautious concern.
- Early portrayals were often shrouded in mystery, emphasizing their complexity and sophistication. The focus was less on the impact on everyday life and more on the technical aspects of the technology.
- More recently, there has been a greater emphasis on the practical applications and societal implications. Discussions about bias, privacy, and accountability are now more common.
- The evolution reflects the increasing awareness of the technology’s potential impact across various sectors.
Public Awareness and Engagement
Public awareness of neural networks varies significantly. While there is growing understanding, substantial gaps in knowledge remain. Engagement with the technology through educational initiatives, workshops, and public forums can foster a more nuanced understanding.
Summary of Public Perception Evolution
Time Period | Public Perception | Examples |
---|---|---|
Early 2010s | Neural networks were a relatively obscure technology. | Media coverage focused on research breakthroughs, with limited discussion of wider implications. |
Mid-2010s | Increasing awareness of applications in various fields, like image recognition and natural language processing. Positive narratives were prominent. | Articles highlighting advancements in medical diagnosis and self-driving cars. |
Late 2010s to Present | Growing awareness of potential risks and ethical concerns. Discussions about bias, privacy, and job displacement became more common. | News reports on algorithmic bias in loan applications, debates about autonomous weapons systems. |
Challenges and Future Trends
Neural networks are rapidly moving into the mainstream, promising revolutionary advancements across various sectors. However, this surge in adoption brings forth significant challenges that must be addressed for the technology to truly flourish and benefit society. Understanding these hurdles and future directions is crucial for harnessing the full potential of neural networks.The path to widespread adoption is paved with both exciting possibilities and substantial obstacles.
Data privacy and security concerns are paramount, requiring robust solutions. The increasing complexity of neural networks also presents challenges for interpretability and trust, demanding rigorous research and development in explainable AI. Moreover, the substantial computational resources required for training and deploying these models pose barriers for smaller organizations and researchers.
Challenges to Widespread Adoption
Several factors hinder the broader application of neural networks. Computational demands are substantial, requiring powerful hardware and specialized expertise. The need for large, high-quality datasets is another critical challenge. Developing robust algorithms and models that are accurate, reliable, and resistant to adversarial attacks is essential for mainstream acceptance.
Concerns Regarding Data Privacy and Security
Data privacy and security are paramount concerns in the context of neural network applications. Neural networks often operate on vast datasets, raising concerns about the protection of sensitive personal information. The potential for data breaches and misuse of personal data must be addressed with strong data governance and security protocols. Furthermore, the training data itself can reflect and perpetuate existing biases, potentially leading to discriminatory outcomes in applications like loan approvals or hiring processes.
Careful consideration and mitigation strategies are necessary to prevent these issues.
Future Trends and Directions
Several future trends are shaping the evolution of neural network technology. The development of explainable AI (XAI) is crucial for building trust and understanding in the decision-making processes of neural networks. Federated learning, which allows for training models on decentralized datasets, is emerging as a solution to address data privacy concerns. Edge computing, where neural network computations are performed closer to the data source, can reduce latency and improve efficiency.
Neural network technology is finally hitting the mainstream, and that’s a huge deal. This surge in popularity is intertwined with the need for a robust internet infrastructure, which is why the future internet protocol, IPv6, is poised for adoption. As more devices connect and the complexity of neural networks grows, a smooth transition to IPv6 is essential for the continued growth of this technology.
The shift to IPv6 is crucial for the long-term success of neural network technology. internet protocol for the future ipv6 poised for adoption is a key factor in making this possible.
Predictions for Impact on the Future
Neural networks are poised to transform numerous aspects of our lives. In healthcare, they can aid in disease diagnosis and personalized treatment plans. In finance, they can enhance fraud detection and risk assessment. In transportation, they are driving advancements in self-driving vehicles. These are just a few examples of the profound impact neural networks will have on our future.
Table of Predicted Future Applications and Challenges
Application | Predicted Impact | Potential Challenges |
---|---|---|
Personalized Medicine | Improved diagnosis, targeted treatments, and enhanced patient outcomes. | Data privacy concerns, algorithmic bias, and the need for rigorous validation. |
Autonomous Vehicles | Enhanced safety, reduced traffic congestion, and improved efficiency in transportation. | Ensuring reliability and safety in complex environments, addressing ethical concerns, and overcoming regulatory hurdles. |
Financial Fraud Detection | Reduced financial losses due to fraud, enhanced security, and improved risk assessment. | Data security, potential for algorithmic bias, and maintaining real-time responsiveness. |
Climate Modeling | Improved climate predictions, enabling better resource management, and promoting sustainable practices. | Computational demands, data availability, and the need for robust validation methods. |
Case Studies and Examples
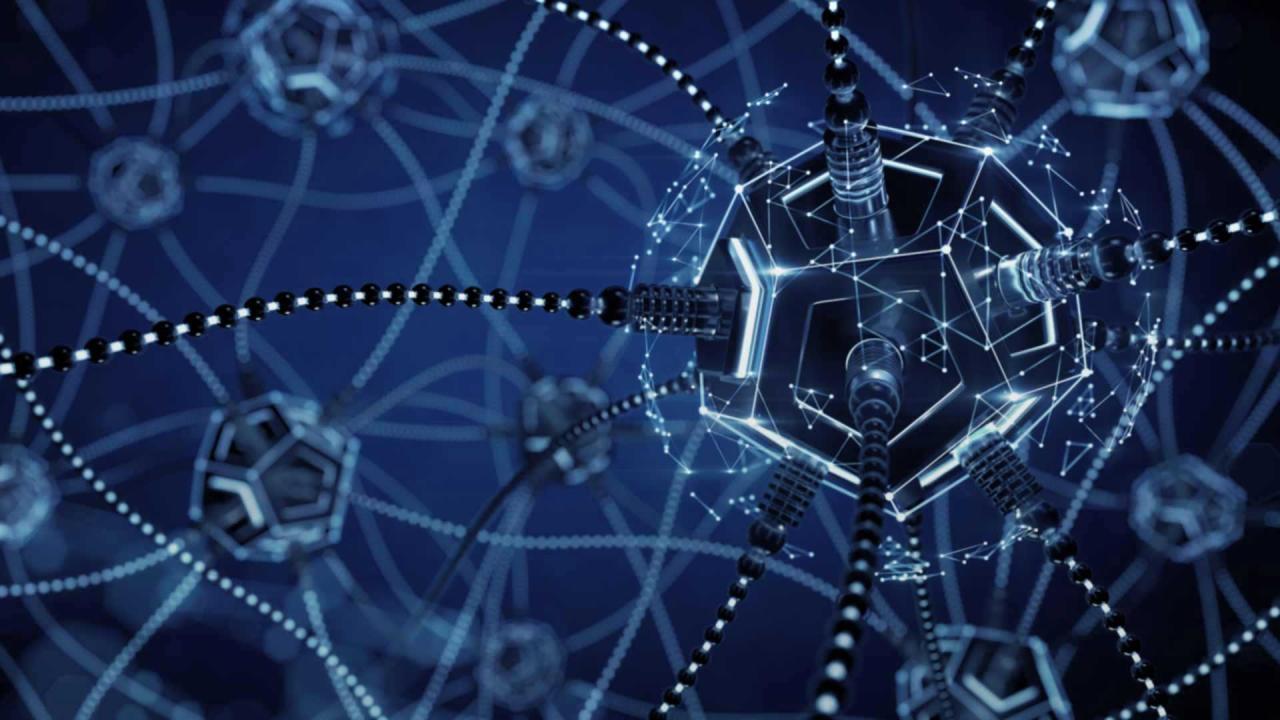
Neural networks are no longer confined to the realm of science fiction; they’re increasingly woven into the fabric of our daily lives. From recommending products online to diagnosing medical conditions, their impact is undeniable. This section explores practical applications, demonstrating how neural networks are reshaping industries and improving everyday experiences.This section provides concrete examples of neural network applications, highlighting their success in various fields.
We will look at how they are used in diverse sectors, from finance to healthcare, to illustrate their transformative potential. The goal is to move beyond abstract concepts and show the tangible results of these powerful technologies.
Neural network technology is finally hitting the mainstream, impacting everything from image recognition to natural language processing. This surge in adoption is directly fueling the rise of socially intelligent software agents, like those discussed in this insightful piece on socially intelligent software agents go mainstream. The increased capability and accessibility of neural networks are making these agents more practical and widespread, pushing the boundaries of what’s possible in AI and further solidifying the mainstream acceptance of neural network technology.
Successful Applications in Finance
Neural networks are revolutionizing financial services, enhancing accuracy and efficiency in areas like fraud detection and risk assessment. Sophisticated algorithms can analyze vast datasets of financial transactions to identify patterns indicative of fraudulent activity, significantly improving security and reducing financial losses.
Neural network technology is finally hitting the mainstream, making its way into everyday devices and applications. This progress is mirrored by advancements in storage, like Lexar’s new 4 GB CompactFlash card, which promises faster data transfer speeds. These developments show how interconnected technological progress is, with both areas benefiting from similar improvements in speed and efficiency, pushing the boundaries of what’s possible in both fields.
- Fraud Detection: Banks and financial institutions are employing neural networks to identify suspicious transactions in real-time. By analyzing transaction patterns, network anomalies, and customer behavior, these systems can flag potential fraud with high accuracy, minimizing the risk of unauthorized access and financial loss.
- Algorithmic Trading: High-frequency trading firms leverage neural networks to make split-second trading decisions. These systems analyze market trends, news sentiment, and other data points to identify opportunities for profitable trades. This automated approach can significantly increase trading speed and efficiency, though it also presents potential challenges related to market manipulation.
Applications in Healthcare
The impact of neural networks in healthcare is substantial, assisting in diagnosis, drug discovery, and personalized medicine. Their ability to process complex medical images and data allows for faster and more accurate diagnoses, leading to better patient outcomes.
- Medical Image Analysis: Neural networks excel at analyzing medical images like X-rays, CT scans, and MRIs. They can detect subtle anomalies that might be missed by human experts, potentially leading to earlier diagnoses and improved treatment plans.
- Drug Discovery: Neural networks can accelerate the drug discovery process by analyzing vast datasets of molecular structures and biological information. This allows researchers to identify potential drug candidates more efficiently and potentially reduce the time and cost required to bring new medications to market.
Everyday Applications of Neural Networks
Neural networks are becoming increasingly prevalent in our daily routines, often without our conscious awareness.
- Recommendation Systems: Platforms like Netflix, Amazon, and Spotify utilize neural networks to personalize recommendations. By analyzing user preferences and viewing history, these systems predict what content or products a user might enjoy, increasing user engagement and satisfaction.
- Natural Language Processing (NLP): Neural networks are crucial for enabling applications like spam filtering, language translation, and chatbots. These technologies use complex algorithms to understand and respond to human language, making interactions with technology more intuitive and user-friendly.
Disruptive Use Cases and Industry Disruption
Neural networks are disrupting various industries by automating tasks, improving efficiency, and generating new revenue streams.
- Customer Service Chatbots: Many companies are deploying chatbots powered by neural networks to handle customer inquiries. These automated systems can provide instant support, answer common questions, and even resolve simple issues, freeing up human agents for more complex tasks.
- Autonomous Vehicles: Neural networks are a fundamental component of self-driving cars, enabling them to perceive their surroundings, make decisions, and navigate safely. The use of these systems is paving the way for a future of safer and more efficient transportation.
Accessibility and Inclusivity
Neural networks are rapidly transforming various sectors, but their true potential hinges on ensuring equitable access and inclusivity. This requires careful consideration of how these powerful tools can be made available to diverse communities and how they can address societal challenges. Ignoring these factors risks exacerbating existing inequalities and hindering the widespread benefits of this technology.Addressing accessibility and inclusivity in neural network technology is crucial for fostering a more equitable and just future.
This involves not only making tools accessible but also ensuring that the technologies themselves are developed with diverse needs in mind, so that their impact benefits everyone, not just a select few. This approach requires collaboration between technologists, educators, policymakers, and community representatives to ensure that everyone can benefit from this transformative technology.
Analyzing Accessibility of Neural Network Tools
Neural network tools, while powerful, are often complex and require specific technical expertise to operate. This can create barriers for individuals and communities lacking access to education and resources. Efforts to improve accessibility involve user-friendly interfaces, simplified training processes, and the development of accessible documentation and support materials.
Using Neural Networks to Address Societal Issues
Neural networks can play a crucial role in addressing a wide range of societal challenges. For instance, they can be employed in healthcare to improve diagnostic accuracy and personalize treatment plans. In education, they can tailor learning experiences to individual student needs, potentially closing achievement gaps. Beyond these applications, neural networks are increasingly used in areas such as poverty alleviation and disaster relief, demonstrating their versatility in tackling pressing social problems.
Role of Education and Training
Education and training are essential for fostering broader adoption of neural network technology. Comprehensive programs should equip individuals with the necessary skills and knowledge to understand, utilize, and contribute to the development of these technologies. These programs should be adaptable to various learning styles and accommodate diverse backgrounds and skill levels.
Access Levels and Training Opportunities
Community | Access Level | Training Options |
---|---|---|
Low-income communities | Limited access to high-speed internet and computing resources. | Online courses with affordable or free options, community workshops in accessible locations, and partnerships with local libraries and community centers. |
Individuals with disabilities | Need for accessible interfaces, tools, and support. | Specialized training programs that incorporate assistive technologies, user-friendly interfaces, and adaptive learning platforms. |
Underrepresented groups (e.g., women, minorities) | May face bias in algorithms and lack of representation in development teams. | Mentorship programs, scholarships, and initiatives to promote diversity and inclusion in AI education and development. |
Rural communities | Limited access to specialized training and resources. | Mobile training programs, remote access to online courses, and partnerships with local colleges and universities. |
Conclusion: Neural Network Technology Moves Into The Mainstream
In conclusion, the mainstream adoption of neural network technology is a significant development with far-reaching implications. The integration of these powerful tools into various sectors has led to remarkable advancements and innovations. However, challenges such as data privacy and ethical considerations must be addressed alongside continued advancements in the technology. The future holds exciting possibilities for neural networks, and it’s crucial to navigate this new landscape responsibly and thoughtfully.