Neurotech Links Brain Waves with Computers A Deep Dive
Neurotech links brain waves with computers, opening a fascinating window into the potential of direct brain-computer communication. This exploration delves into the history, methods, and applications of this revolutionary technology, promising to reshape healthcare, communication, and even entertainment. From early attempts to interpret brain signals to the complex algorithms used today, we’ll examine the entire process, exploring the ethical implications along the way.
The core of this technology lies in understanding how our brains generate electrical signals, and how these signals can be captured, processed, and ultimately translated into commands or outputs. This journey will uncover the exciting possibilities and potential challenges inherent in this burgeoning field.
Introduction to Neurotech
Neurotechnology, encompassing the intersection of neuroscience and technology, is rapidly evolving, promising to revolutionize healthcare, communication, and human-computer interaction. This field focuses on understanding the intricate workings of the brain and developing tools to interact with it, ultimately aiming to improve human capabilities and treat neurological disorders. From basic research to real-world applications, neurotechnology is poised to reshape our understanding of the mind and its potential.The historical development of brain-computer interfaces (BCIs) demonstrates a gradual progression from theoretical concepts to tangible devices.
Early attempts, often reliant on crude measurements of brain activity, laid the groundwork for today’s more sophisticated technologies. This journey underscores the continuous refinement and technological advancement required to translate basic scientific discoveries into clinically relevant applications.
Historical Context of Brain-Computer Interfaces
Early attempts at interpreting brain signals predate modern neuroimaging techniques. Pioneering researchers, driven by a desire to understand the neural basis of consciousness and behavior, experimented with various methods to detect electrical activity in the brain. For instance, some early researchers explored the relationship between muscle contractions and electrical potentials in the brain. These early studies, although limited in scope and precision, provided crucial insights into the possibility of communication between the brain and external devices.
Fundamental Principles of Brain-Computer Interfaces
Brain-computer interfaces rely on the principle that brain activity, in the form of electrical signals, can be detected and translated into commands or information that can be interpreted by a computer. Electroencephalography (EEG), magnetoencephalography (MEG), and electrocorticography (ECoG) are common methods for recording brain signals. These techniques measure different aspects of neural activity, from macroscopic patterns of electrical activity to the localized activity of individual neurons.
The translation of these signals into meaningful outputs requires sophisticated signal processing algorithms.
Examples of Early Attempts
Early efforts to interpret brain signals often focused on basic sensory and motor functions. One notable example is the study of the relationship between brain activity and muscle movements. Researchers attempted to identify specific patterns in brain activity that corresponded to specific motor commands. These studies provided early insights into the potential of BCIs for controlling external devices.
Key Components of a Typical Brain-Computer Interface
Component | Description |
---|---|
Signal Acquisition | This involves the process of detecting and recording brain signals using techniques like EEG, MEG, or ECoG. |
Signal Processing | This crucial step involves filtering, amplifying, and analyzing the raw brain signals to extract meaningful patterns and information. Signal processing algorithms identify and interpret relevant features within the complex signals. |
Feature Extraction | This process identifies specific characteristics of the brain signals that correlate with the desired output or action. |
Classification/Decoding | Algorithms are trained to classify these extracted features and translate them into specific commands or outputs. |
Output Device | The system interfaces with the desired output, which could range from a computer cursor to a prosthetic limb. |
Methods of Brain Signal Acquisition
Neurotechnology is rapidly advancing, allowing us to delve deeper into the intricate workings of the human brain. A crucial aspect of this exploration lies in the methods used to capture and interpret brain signals. Different techniques offer varying levels of detail and resolution, each with unique strengths and weaknesses. Understanding these methods is essential for appreciating the capabilities and limitations of neurotech applications.
Electroencephalography (EEG), Neurotech links brain waves with computers
EEG measures electrical activity in the brain by placing electrodes on the scalp. The electrical signals, reflecting the summed activity of millions of neurons, are amplified and recorded. This technique is non-invasive, relatively inexpensive, and provides excellent temporal resolution, meaning it can track brain activity in real-time. However, EEG has limited spatial resolution, making it challenging to pinpoint the exact location of the source of activity within the brain.
The signal is also easily influenced by external factors, such as eye movements and muscle activity, requiring careful experimental design and data processing.
Magnetoencephalography (MEG)
MEG measures magnetic fields generated by electrical activity in the brain. Superconducting quantum interference devices (SQUIDs) detect these minute magnetic fields, which are less susceptible to noise than EEG signals. MEG offers excellent temporal resolution, comparable to EEG, and better spatial resolution than EEG, allowing for a more precise localization of brain activity. However, MEG is significantly more expensive than EEG and requires a specialized, shielded environment to minimize interference.
Additionally, MEG signals are weaker than EEG signals, which can sometimes limit its sensitivity.
Functional Magnetic Resonance Imaging (fMRI)
fMRI measures changes in blood flow in the brain, which are associated with neural activity. When neurons are active, blood flow to that region increases, providing an indirect measure of brain activity. fMRI offers excellent spatial resolution, enabling the visualization of brain structures and their activity in great detail. It is widely used for mapping brain function and studying brain anatomy.
However, fMRI has limited temporal resolution compared to EEG and MEG, as blood flow changes are slower than neural activity. Also, fMRI measurements are not direct measures of neural activity but rather an indirect proxy for it.
Neurotech is rapidly connecting brain waves to computers, paving the way for incredible advancements. This intricate technology, however, hinges on seamless communication protocols, much like the advancements seen in the Wi-Fi Alliance’s hard work on interoperability standards, as detailed in their latest stance on interoperability. The wifi alliance hardens interoperability stance here highlights the importance of reliable connections in various fields, ultimately impacting the future of neurotech’s ability to translate brain signals into commands or data.
This interconnectivity will be crucial for the continued advancement of neurotech’s potential.
Ethical Considerations
The acquisition of brain signals raises significant ethical considerations. Informed consent is crucial to ensure participants understand the procedures, potential risks, and benefits. Data privacy and security are paramount to protect sensitive information. The potential for misuse of brain signal data for purposes like manipulation or coercion needs careful consideration. Furthermore, equitable access to neurotechnological procedures and data analysis must be ensured.
Neurotech is fascinating, linking brainwaves to computers. This reminds me of the early days of music sharing, like the iTunes phenomenon, P2P networks, and music lite. The ease of access and sharing revolutionized music, and perhaps neurotech will similarly reshape how we interact with and control technology. It’s a wild leap forward, but a truly exciting one for the future of human-computer interaction.
Comparison of Techniques
Technique | Signal Measured | Spatial Resolution | Temporal Resolution | Cost | Invasiveness |
---|---|---|---|---|---|
EEG | Electrical activity | Poor | Excellent | Low | Non-invasive |
MEG | Magnetic fields | Good | Excellent | High | Non-invasive |
fMRI | Blood flow | Excellent | Poor | Medium | Non-invasive |
Acquiring Brainwave Data for a Specific Application: Neurofeedback
A neurofeedback application might involve training individuals to modulate their brainwave activity. The process begins with identifying the specific brainwave patterns to be targeted. Electrodes are placed on the scalp, and brainwave data is continuously recorded. Specialized software analyzes the data in real-time, providing feedback to the participant through visual or auditory cues. For instance, if the participant is asked to reduce their theta wave activity, the software might display a visual reward when theta activity decreases, thus reinforcing the desired response.
Neurotech is fascinating, linking brainwaves to computers, opening up possibilities for amazing advancements. Imagine controlling devices with your mind, a concept that’s becoming more and more real. This technology’s potential is huge, but it’s important to consider the implications of merging human thought with technology, like how the Dell Gateway is now becoming a familiar fixture in many living rooms, thanks to dell gateway settle into the living room.
Ultimately, as neurotech progresses, we’ll need to carefully navigate these new frontiers. This could lead to amazing future applications in areas ranging from medical treatment to gaming.
The training involves repetitive cycles of recording, analysis, and feedback, aiming to establish a learned response associated with the desired brainwave pattern.
Data Processing and Interpretation
Unraveling the secrets hidden within brainwave data requires a meticulous process of data processing and interpretation. Raw brainwave signals, as captured by electrodes, are complex and noisy. Transforming this raw data into meaningful insights about cognitive processes or even predicting future actions necessitates a series of steps that carefully filter, analyze, and interpret the patterns within the signal.
This process, while complex, is crucial for extracting valuable information and enabling the development of neurotech applications.
Steps in Processing Raw Brainwave Data
The journey from raw EEG signals to actionable insights involves several crucial steps. These steps are designed to filter out noise, isolate specific brainwave patterns, and prepare the data for further analysis.
- Signal Preprocessing: Raw brainwave data often contains unwanted noise from various sources, including electrical interference, eye movements, and muscle activity. Preprocessing techniques, such as filtering, artifact rejection, and baseline correction, are essential to remove this noise and isolate the brain’s true signal. Sophisticated algorithms are employed to identify and remove artifacts, improving the signal’s quality and accuracy.
- Signal Segmentation: Brainwave data is often segmented into smaller, manageable chunks for analysis. This segmentation can be based on specific time intervals, events, or task periods. This approach allows for more focused analysis of specific cognitive activities or states.
- Feature Extraction: The extracted segments are then analyzed to identify specific features. These features, such as amplitude, frequency, and phase, characterize the brainwave patterns. This step is critical for distinguishing different types of brain activity and for later classification tasks.
Algorithms for Interpreting Brain Signals
Various algorithms are used to interpret the extracted features from brainwave data. These algorithms are essential for identifying patterns and correlating them with specific cognitive processes.
- Machine Learning Algorithms: Machine learning algorithms, such as support vector machines (SVM), artificial neural networks (ANNs), and deep learning models, are frequently used to analyze brainwave patterns. These algorithms are trained on labeled datasets to learn the relationships between brainwave patterns and corresponding cognitive states. This training allows the algorithms to classify different brain states, predict user intentions, or identify specific cognitive processes.
For example, an ANN trained on EEG data from subjects performing a task can predict the subject’s next action with a high degree of accuracy.
- Time-Frequency Analysis: Time-frequency analysis techniques, like wavelet transforms, allow the identification of brainwave activity at different frequencies and time points. This method is useful in understanding the dynamic nature of brain activity, as it allows researchers to examine how the frequency content of brainwaves changes over time.
- Statistical Analysis: Statistical methods, including statistical significance tests and correlation analysis, are used to identify statistically significant relationships between brainwave patterns and behavioral or cognitive variables. These methods help researchers determine whether observed correlations are likely due to chance or reflect a genuine relationship.
Challenges in Extracting Meaningful Information
Despite the advancements in neurotechnology, several challenges persist in extracting meaningful information from brainwave patterns.
- Inter-individual Variability: Brainwave patterns vary significantly between individuals, making it challenging to establish generalizable patterns or models. Individual differences in brain anatomy, physiology, and cognitive styles contribute to this variability. This variability is often accounted for by employing techniques such as normalization.
- Noise and Artifacts: The presence of noise and artifacts in the raw data can significantly affect the accuracy of analysis. Advanced filtering and artifact rejection techniques are needed to minimize the impact of noise on the results.
- Complex Interactions: Brain activity is a complex interplay of various factors, and disentangling the contributions of different neural processes to observed patterns can be difficult. This complexity requires sophisticated analytical approaches.
Transforming Brainwave Data into Actionable Outputs
The ultimate goal of neurotech is to translate brainwave data into actionable outputs. These outputs can range from assistive technologies to cognitive training tools.
- Assistive Technologies: Brainwave data can control assistive devices, such as computer cursors or prosthetic limbs, allowing individuals with limited mobility to interact with their environment. These systems translate brain activity into commands, enabling individuals to perform tasks previously impossible.
- Cognitive Training: Brainwave data can be used to monitor and adapt cognitive training programs, providing personalized feedback and adjustments based on the user’s brain activity. This approach can optimize learning and improve cognitive performance.
- Healthcare Applications: Brainwave analysis can be used to diagnose neurological disorders, monitor patient responses to treatment, and potentially predict future health risks. This approach holds immense promise for improving patient outcomes and healthcare practices.
Flowchart of Data Interpretation
A typical flowchart for interpreting brainwave data begins with the raw data and follows a series of steps, including preprocessing, segmentation, feature extraction, analysis, and output generation. The flowchart visually depicts the sequential processing of the data. The specific algorithms and techniques used will depend on the specific application and goals.
[Imagine a simple flowchart here. It would show a process starting with “Raw EEG Data” and branching to “Preprocessing,” “Segmentation,” “Feature Extraction,” “Algorithm Application,” and “Interpretation/Output.” Each box would be connected by arrows, indicating the flow of the process.]
Applications of Neurotech Links: Neurotech Links Brain Waves With Computers
Neurotechnology, bridging the gap between the human brain and computers, holds immense potential for transforming various aspects of our lives. From enhancing communication to improving healthcare and even revolutionizing entertainment, the possibilities are vast and rapidly expanding. This section explores the diverse applications of neurotech, highlighting its impact on various sectors.
Real-World Applications
Neurotechnology is already being applied in numerous real-world scenarios. One significant area is the control of prosthetic limbs. Patients with paralysis can now potentially use their brain activity to control robotic limbs, restoring a degree of independence and functionality. Similarly, neurotech is being used to assist individuals with communication challenges, enabling them to interact with the world through brain-computer interfaces (BCIs).
Improving Healthcare
Neurotechnology offers exciting possibilities for enhancing healthcare. Early detection and diagnosis of neurological disorders are becoming increasingly feasible with neurotech. By monitoring brain activity, doctors can identify patterns indicative of conditions like Alzheimer’s or Parkinson’s disease in their early stages, potentially enabling earlier intervention and more effective treatments. Furthermore, neurotech can aid in personalized therapies, tailoring treatment plans based on individual brain responses.
Neurotech in Gaming and Entertainment
The gaming and entertainment industries are exploring the potential of neurotech to create immersive and interactive experiences. Players could potentially control game elements with their thoughts, leading to more responsive and intuitive gameplay. Imagine controlling a character’s actions or manipulating game objects by simply thinking about them. This opens up new avenues for creating highly engaging and personalized entertainment experiences.
Neurotech Applications Table
Application | Benefits |
---|---|
Prosthetic Control | Improved mobility and independence for individuals with paralysis or limb loss. |
Communication Assistance | Enhanced communication for individuals with speech or motor impairments. |
Healthcare Diagnosis | Early detection of neurological disorders, personalized therapies, and improved treatment outcomes. |
Gaming and Entertainment | Enhanced user experience, more intuitive control, and creation of immersive experiences. |
Medical Scenario Example: Epilepsy Treatment
In the case of epilepsy, neurotech can be applied through implantable devices that monitor brain activity in real-time. These devices can detect patterns associated with seizures and trigger preventative measures, such as administering medication or alerting medical personnel. Furthermore, these devices can aid in identifying the specific brain regions responsible for seizures, enabling more targeted and effective surgical interventions.
The specific procedures involve the surgical implantation of electrodes, signal processing to identify seizure patterns, and the integration of the system with a monitoring and response system. Careful consideration of ethical implications and patient safety is crucial throughout this process.
Future Directions and Possibilities
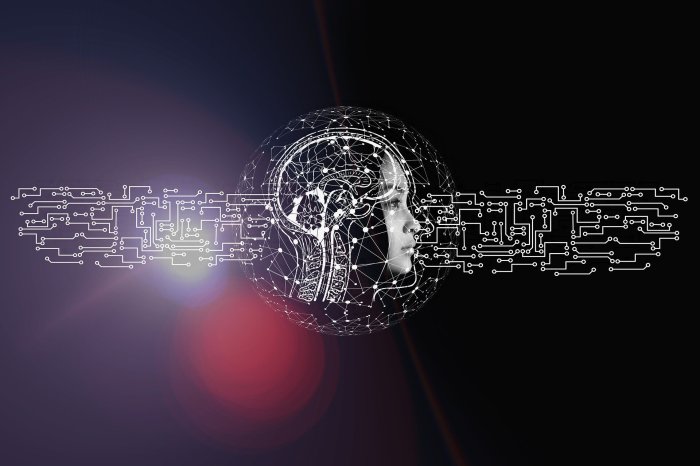
Neurotechnology’s potential to bridge the gap between the human brain and computers is poised for significant advancement. Current research focuses on refining signal acquisition, processing, and interpretation techniques, laying the groundwork for more sophisticated and practical applications. The future promises a convergence of neuroscience, engineering, and computer science, leading to breakthroughs in areas from medical diagnostics to human-computer interaction.The emerging field of neurotechnology is rapidly expanding, driven by innovative approaches and the ever-increasing computational power available.
This advancement promises to transform various aspects of human life, offering exciting possibilities for improving human well-being and understanding the intricate workings of the mind.
Potential Breakthroughs in Brain-Computer Interfaces
Advancements in brain-computer interfaces (BCIs) are expected to lead to more intuitive and efficient methods of communication and control. Research is focusing on developing BCIs that can decode complex cognitive processes, such as thoughts and emotions, with greater accuracy. This could pave the way for more natural and seamless interaction between humans and machines, revolutionizing fields like assistive technology and rehabilitation.
For instance, imagine a paralyzed individual controlling a robotic arm or a prosthetic limb solely through their thoughts, allowing them greater independence and autonomy.
Emerging Trends in Neurotech Applications
Several emerging trends are shaping the future of neurotechnology. One significant trend is the integration of AI and machine learning algorithms into BCI systems. These algorithms can analyze brain signals with increasing sophistication, enabling more accurate and reliable decoding of intentions and emotions. Another trend is the development of non-invasive BCI technologies, such as electroencephalography (EEG) and functional near-infrared spectroscopy (fNIRS).
These methods offer less invasive approaches to studying brain activity, making them more accessible and less risky for research and clinical use.
Societal Impact of Widespread Neurotech Adoption
The widespread adoption of neurotechnology will undoubtedly have profound societal impacts. Positive impacts include improved healthcare outcomes through early disease detection and personalized treatments. Furthermore, neurotech applications could enhance human cognitive abilities and performance, potentially leading to greater productivity and innovation in various sectors. However, potential negative impacts also exist, including concerns about privacy, security, and the ethical implications of manipulating or enhancing human cognitive functions.
Different Perspectives on the Future of Neurotech
There are diverse opinions on the future of neurotechnology. Some experts are optimistic about its transformative potential, envisioning a future where neurotech enhances human capabilities and improves lives in profound ways. Others are more cautious, highlighting the potential risks associated with misuse and the need for ethical guidelines and regulations. The debate surrounding the future of neurotech is complex and multifaceted, involving both potential benefits and concerns.
Ethical Concerns and Proposed Solutions
Ethical Concern | Proposed Solution |
---|---|
Privacy of brain data | Establishing strict data security protocols and regulations, anonymizing data whenever possible, and providing informed consent mechanisms for data collection. |
Potential for misuse | Developing clear ethical guidelines and regulations for the development and use of neurotech, establishing oversight committees and mechanisms for accountability. |
Accessibility and equity | Promoting equitable access to neurotech applications and ensuring that the benefits of these technologies are distributed fairly across different socioeconomic groups. |
Impact on human identity and autonomy | Engaging in ongoing public discourse and ethical deliberation on the potential impact of neurotech on human identity and autonomy, fostering a multidisciplinary approach to addressing the complex issues involved. |
Bias in algorithms | Implementing rigorous testing and validation procedures for neurotech algorithms to minimize biases and ensure fair and equitable outcomes. |
Illustrative Examples
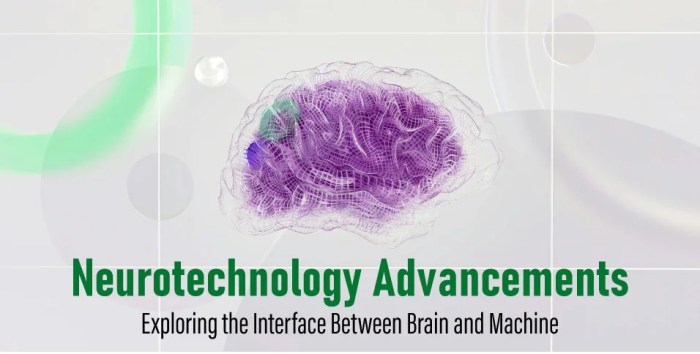
Neurotechnology is rapidly advancing, and brain-computer interfaces (BCIs) are becoming increasingly sophisticated. These interfaces promise to revolutionize human interaction with technology, enabling unprecedented levels of control and communication. Understanding the practical applications and potential of BCIs requires exploring illustrative examples, demonstrating how these technologies are being implemented and what they can achieve.These examples provide tangible insights into the capabilities of BCIs, highlighting their potential to address medical needs and enhance human capabilities.
We’ll examine a scenario of a patient controlling a robotic arm, visualize a BCI’s structure, and delve into the neural pathways involved in a specific application. We’ll also trace the steps involved in developing a BCI and show how brainwaves interact with computers.
Patient Controlling a Robotic Arm
A patient with severe motor impairments, perhaps due to a spinal cord injury, could use a BCI to control a robotic arm. Electrodes implanted in the motor cortex, the brain region responsible for voluntary movement, detect the patient’s intended movements. These electrical signals are then processed by an algorithm that translates the brain activity into commands for the robotic arm.
The patient can thus manipulate objects, perform tasks, and regain a degree of independence.
Visual Representation of a Brain-Computer Interface
Imagine a simplified representation of a BCI as a series of interconnected components. A cap fitted with electrodes rests on the scalp. These electrodes detect electrical activity generated by neurons in the brain. The signals are then amplified and processed by a signal processing unit. This unit converts the raw brain signals into meaningful commands.
These commands are then sent to a computer which in turn interacts with the robotic arm, executing the movement. A visual representation of this system could show the brain as the source, the electrodes as a bridge, the processing unit as the translator, and the robotic arm as the effector.
Neural Pathways in Robotic Arm Control
The neural pathways involved in controlling a robotic arm are complex. When a patient intends to move their arm, signals from the motor cortex travel down the spinal cord, triggering muscle contractions. With a BCI, this process is bypassed. The patient’s intention is directly detected by the electrodes, processed, and translated into commands for the robotic arm.
The brain’s motor commands are effectively “decoded” by the BCI and relayed to the robotic arm, enabling movement without the need for the usual spinal cord pathway.
Steps in Creating a BCI for Robotic Arm Control
Developing a BCI for robotic arm control involves several crucial steps. First, precise electrode placement is essential to accurately capture neural signals related to arm movement. Second, sophisticated signal processing algorithms are developed to decipher the intended movements from the brain activity. Third, calibration is critical; the system must learn the patient’s unique neural patterns. Finally, the system must be tested for reliability and safety in real-world scenarios.
Diagram of Brainwave-Computer Connection
Component | Description |
---|---|
Brain | Generates electrical signals representing intended actions. |
Electrodes | Detect electrical signals from the brain. |
Signal Processing Unit | Amplifies, filters, and interprets brain signals into commands. |
Computer | Receives and processes the commands. |
Robotic Arm | Executes the commands received from the computer. |
A diagram depicting this process would show a simplified representation of the brain, electrodes attached to the scalp, lines representing signal transmission to a processing unit, and finally, the connection to the robotic arm.
Final Summary
In conclusion, neurotech’s ability to link brain waves with computers represents a significant leap forward in human-machine interaction. From controlling prosthetic limbs to aiding communication for those with disabilities, the applications are vast and transformative. While ethical considerations and technical hurdles remain, the future of this technology holds immense promise, potentially revolutionizing many aspects of our lives. The detailed exploration of the underlying mechanisms, diverse applications, and potential future developments presented here offers a comprehensive view of this exciting field.